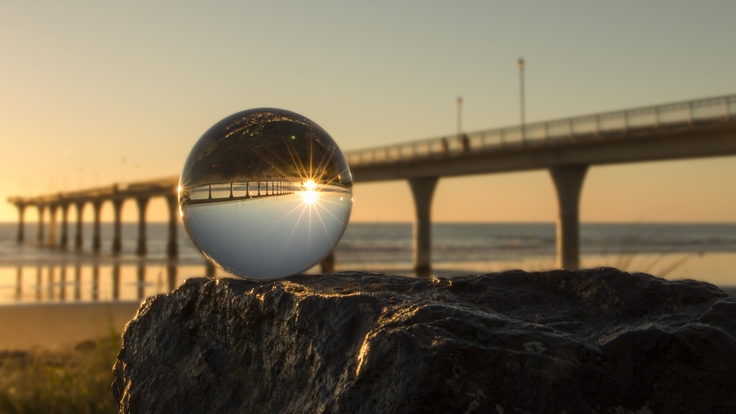
The SingleStore maturity framework for data warehousing in the age of artificial intelligence depicts the journey of digital transformation.
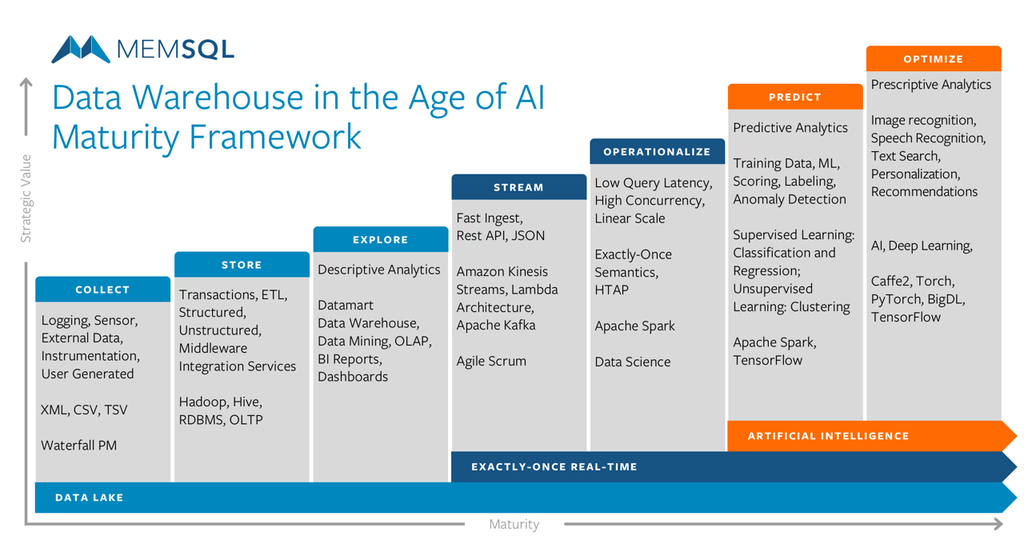
Consisting of three epics and seven maturation stages that symbolize increasing strategic value, the framework outlines the transformation from descriptive analytics to predictive analytics to prescriptive analytics for modern organizations and enterprises.
In this blog post, we characterize each epic and corresponding stages of maturation in detail.
Data Lake

The dark days of largely spreadsheet-based analyses are fading. Opportunistic departments and business units prefer to pursue their own analytics and business intelligence initiatives.
While most organizations have a strong ability to collect and store historical data that is transactional in nature, they continue to endure the hardship of turning data into meaningful analysis.
We have a 100 TB data lake. We don’t know what’s really in it. It’s that big.— Big Data operations team
To help alleviate the burden of transforming data into analytics at larger organizations, leadership governs data with shared services, technology standards, and common data models. Here, key performance indicators characterize descriptive analytics for decision making.
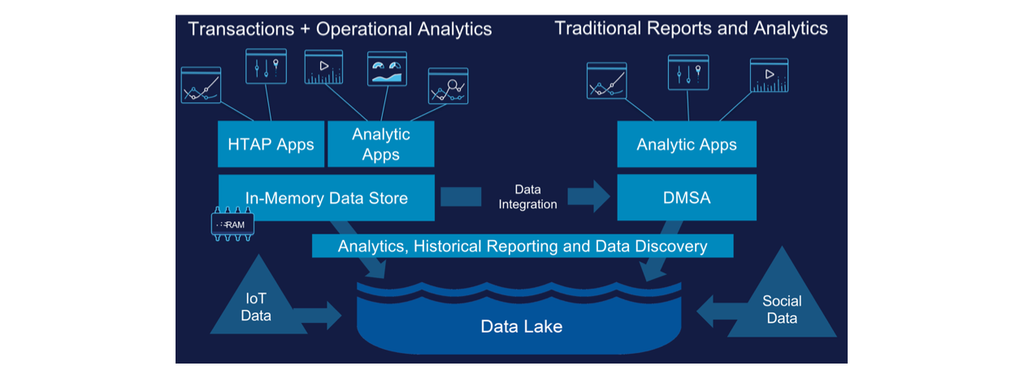
Collect

Row-based and hierarchical data files are a routine matter for various types of data collection. Data includes logs, instrumentation, messaging, user-generated events, and application programming interfaces. The focus on data collection overshadows the initiative to examine the data. Typically, a waterfall project management methodology steers data collection.
Store

General practices for managing enterprise data volume and variety exist in the enterprise: relational databases manage structured application transactions, and NoSQL databases manage document and unstructured data applications.
Batch processes collect and transform bulk data into pre-aggregated cubes defined by rigid snowflake and star schemas inherent to data marts and data warehouses. Disjointed technologies create some bottlenecks that hinder the workflow of turning raw data into business metrics.
Big data collection and storage often consists of a hodgepodge of technology solutions strung closely together. Disparate in-house, open source, and commercial solutions assist with both extraction, transformations, and load (ETL) processes as well as middleware integrations services. Missing automation between the various steps of data ingestion, ETL, and data modeling create noticeable productivity inefficiencies. When one system fails, internal operators scramble to triage the domino chain of failures.
Explore

On a routine basis, individuals share ad-hoc analytical insights. Monthly, weekly, and daily cadences set the standard for departmental reporting. Business intelligence (BI) dashboards offer business users canned reporting, one-off analyses, and ad-hoc reports. Common BI tools include Looker, Tableau, and Zoomdata.
The siloed roles of a data engineer, data scientist, and business user often independently explore and mine data to solve business problems. Some cross-collaboration occurs for standardizing descriptive metrics associated with key performance indicators of various business units. Internally, data teams struggle with how the greater organization properly values the descriptive metrics embedded in data reporting and BI dashboards. Difficult cross-functional collaboration, data veracity suspicions, or misaligned distrust in analytics impairs business innovation.
Exactly-Once Real-Time

For many organizations, real-time data processing means mastering the transactional semantics of exactly-once for data volume, variety, and velocity.
By definition, the always-on economy requires real-time analytics.
— Raghunath Nambiar, Chief Technology Officer Cisco UCS
Legacy messaging systems for real-time data either send ‘At Most Once’, failing to guarantee message receipt, or ‘At Least Once’, promising the guarantee of a receipt at the expense of message duplication.
When these systems experience high data velocity and volume, the pain of duplicate and erroneous data amplifies logarithmically, generating data distrust and excessive post-processing. For many NoSQL solutions, streaming data stops here, waiting to be re-processed and de-duplicated.
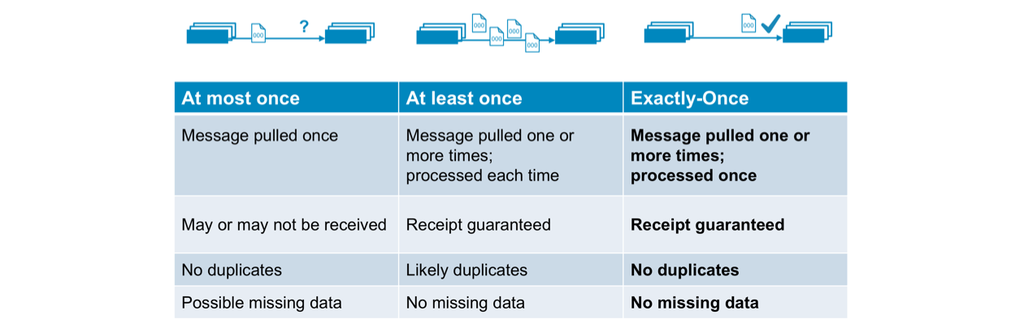
Modern messaging services such as Apache Kafka stream huge volumes at high velocity. The semantics of ‘Exactly-Once’ ensures data receipt without message duplication and error.
Enterprises that harness real-time data marry it with historical data in real-time data warehouses. The data marriage represents operationalized unification, often reflecting the leadership-driven transformation of internal cultural values.
Stream

Adherence to agility allows the organization to culturally embrace change and new initiatives more readily. The need to process disparate data as quickly as possible, and at growing scale, highlights data velocity as a modern requirement of sensor, geospatial, and social media data-driven applications.
Real-time messaging technologies such as Amazon Kinesis and Apache Kafka introduce new found semantic challenges for transactional boundaries associated with microsecond data ingest. Data infrastructure teams struggle to maintain complex data infrastructures that span on-premises and cloud environments. Operations and infrastructure teams start to invest in technologies that accommodate ultrafast data capture and processing. Teams focus on in-memory data storage for high-speed ingest, distributed and parallelized architectures for horizontal scalability, and queryable solutions for real-time, interactive data exploration.
Operationalize

Recognizing that familiar data integration patterns centered on batch processing of bulk data function to disable modern digital business processes, organizations turn to leadership for data governance, unification, and innovation.
Rather than solely standardizing on common data models, Chief Data Officers and Heads of Analytics embrace new topologies for messaging semantics that support exactly-once, real-time data processing. However, leadership moves the proverbial goal post and demands that business value is derived at the time of data ingest.
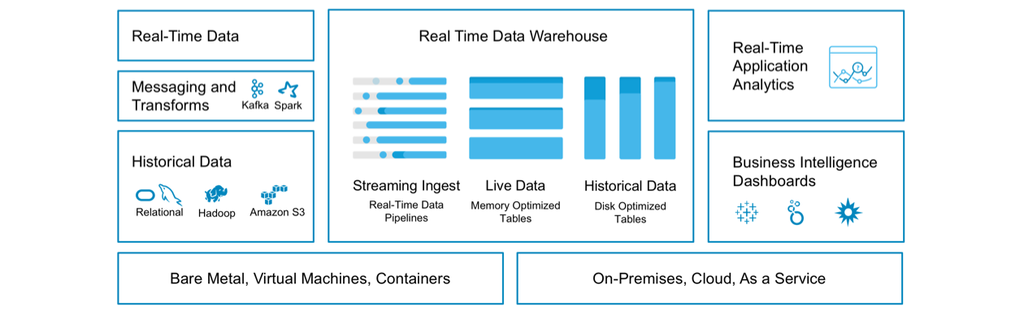
Deriving immediate business value from the moment of data ingest affects architectural decisions and technical requirements. Real-time data processing requires sub-second service level agreements (SLA). As a result, organizations eliminate complex and slow processes for data sanitization including de-duplication. Achieving sub-second business value means maximizing compute cycles while mitigating the slowness associated with traffic spikes and network outages. In sub-second speed, data pipelines operationalize time-to-value.
Keeping low latency SLAs under high concurrency at petabyte volume, necessitates adopting distributed systems for parallelized compute and storage at linear scale. Leadership tasks data engineers, applications developers, and operations teams to implement hybrid transactional and analytical processing in one data platform for real-time and historical data. Collaborative teams identify the anti-pattern: the separation between databases for transactions and data warehouses for analytics.
With a distributed SQL platform, data engineers, data scientists, and business users now can explore and mine data in parallel with other distributed systems for advanced computations such as Apache Spark. Data scientist use Jupyter notebooks to help solve business problems collaboratively. BI dashboards provide business users with real-time reporting of descriptive metrics. Daily intra-day analyses supplant monthly and weekly reporting cadences.
Artificial Intelligence

Enterprises that operationalize historical data from data lakes, and real-time data from messaging streams in a single data platform using a SQL interface, are best positioned to achieve digital transformation.
There is no reason and no way that a human mind can keep up with an artificial intelligence machine by 2035.
— Gray Scott, Futurist & Techno-Philosopher
Even though descriptive metrics can optimize the performance of an organization, the promise of predicting an outcome and recommending the next action is infinitely more valuable. Predictive metrics require training models to learn a process and gradually improve the accuracy of the metric. Deep learning harnesses neural networks for machine learning, creating the ability to simulate intelligence, resulting in specific recommendations.
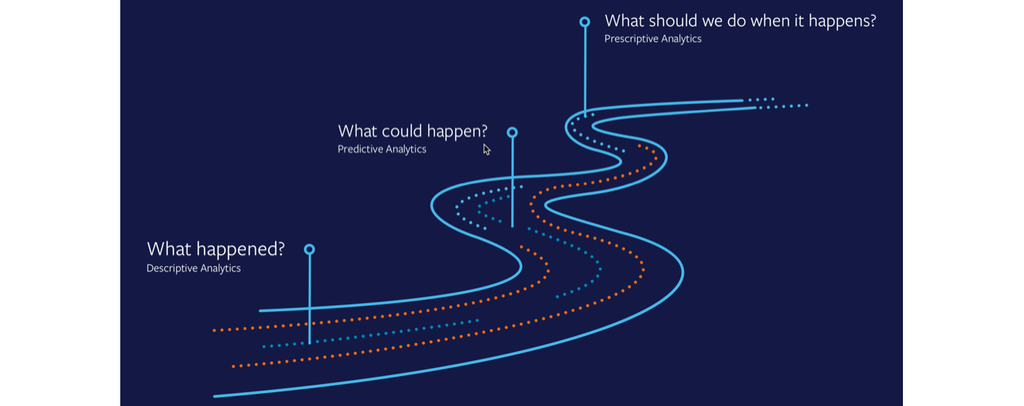
The differences between “What happened?”, “What could happen?”, and “What should we do when it happens?” succinctly illustrates why modern enterprises remain wholly committed to the journey of digital transformation.
Predict

Conventional single-box server systems with fixed computational power and storage capacity, limit machine learning to iterative batch processing. Modern, highly scalable distributed systems for computation, streaming, and data processing, free machine learning to run in real time. Not only can models quickly training on historical data, they operationalize on real-time, streaming data. Continually retraining operationalized models using real-time and historical data together results in optimal tuning.
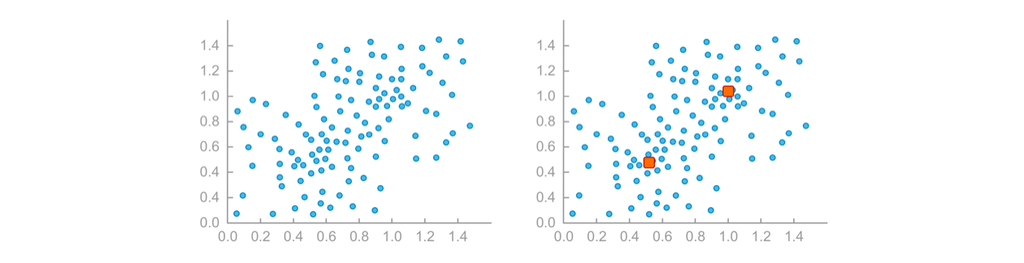
Binary data vectorization allows for distributed, scalable, and parallelized algorithmic processing at ingest. Distributed computational frameworks for CPUs and GPUs such as Apache Spark and TensorFlow easily score streaming data and detect anomalies. The flood gates open for new use cases including real-time event processing, real-time decision making, edge monitoring, churn detection, anomaly detection, and fraud detection.
With the ability to process both real-time and historical data within a single distributed system devoid of external ETL, data governance and management leaders can now own digital transformation initiatives for their organization. The reality of scoring real-time and streaming data with machine learning means that descriptive metrics can now be transformed into predictive analytics.
Optimize

Regression models for machine learning depend on iterative processing. At a certain point, the accuracy of the model prediction levels out or even degrades. Neural network processing for both supervised and unsupervised learning allows for the results of parallel algorithm processing to be recalibrated. Neural network processing with more functional layers of neurons is deep learning. The key to deep learning is allowing for neural network layers to transactionally and incrementally store outputs while evaluating the model for next layer inputs.
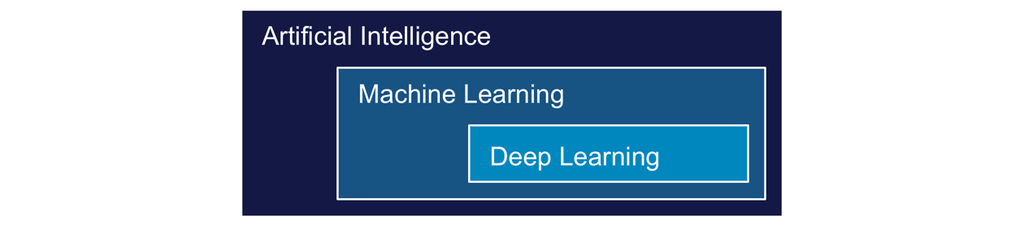
A real-time data warehouse supporting historical data, real-time data ingest, and machine learning, operationalizes deep learning. Implementing standard SQL user-defined functions and stored procedures for machine learning can empower deep learning. With deep learning, predictive analytics generated from closed loop regressions and neural networks turns into prescriptive analytics for real-time recognition, personalization, and recommendations.
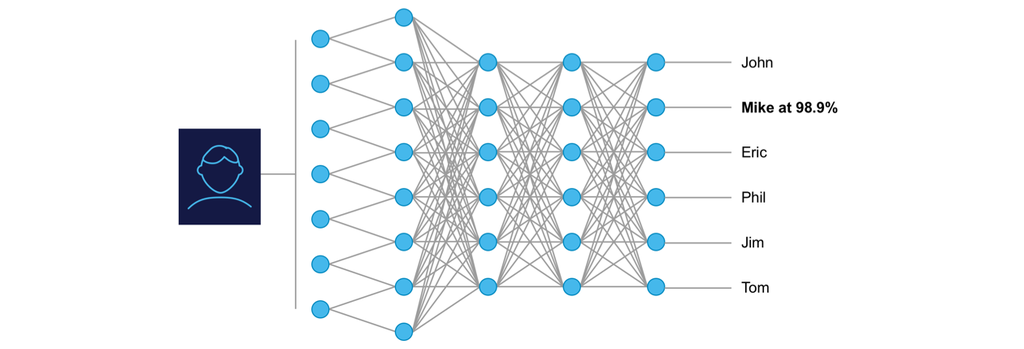
The simplification of algorithmic complexity into procedural language with an SQL interface for artificial intelligence is the foundation for true digital transformation. Optimized organizations evolve from descriptive analytics to predictive analytics to prescriptive analytics. Such organizations gain new found knowledge and competitive advantages about their customers, operations, and competitors with deep context.
Powered by human creativity, the real-time data warehouse represents the exactly-once, AI-powered enterprise with superior abilities to automatically refine accuracy, naturally process sensory perceptions, extrapolate experiences from multiple signal modalities, predict actions, make recommendations, and prescribe results.
Discover Your Journey
In less than 10 minutes, you can start to identify your data warehouse maturity with our free interactive assessment. SingleStore will then generate and share with you, “Your Maturity Framework Interactive Report”.
For many enterprises today, moving to real time is the next logical step, and for some others it’s the only option.
— Nikita Shamgunov, SingleStore co-founder, former CTO, current CEO
Your Maturity Framework Interactive Report
Designed to help your organization determine its current data warehousing maturation level, the report identifies barriers to maturation in the age of artificial intelligence and makes specific recommendations for your next steps.
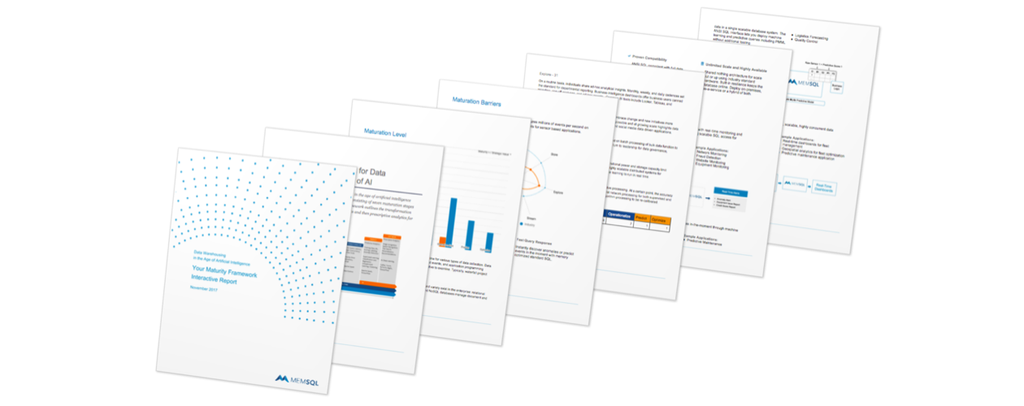
To begin your interactive assessment about your journey, start here.