
Hybrid Search
Notebook

Note
This notebook can be run on a Free Starter Workspace. To create a Free Starter Workspace navigate to Start using the left nav. You can also use your existing Standard or Premium workspace with this Notebook.
Source: OpenAI Cookbook
Hybrid search integrates both keyword-based search and semantic search in order to combine the strengths of both and provide users with a more comprehensive and efficient search experience. This notebook is an example on how to perform hybrid search with SingleStore's database and notebooks.
Setup
Let's first download the libraries necessary.
In [1]:
1
%pip install wget openai==1.3.3 --quiet
In [2]:
1
import json2
import os3
import pandas as pd4
import wget
In [3]:
1
# Import the library for vectorizing the data (Up to 2 minutes)2
%pip install sentence-transformers --quiet3
4
from sentence_transformers import SentenceTransformer5
6
model = SentenceTransformer('flax-sentence-embeddings/all_datasets_v3_mpnet-base')
Import data from CSV file
This csv file holds the title, summary, and category of approximately 2000 news articles.
In [4]:
1
# download reviews csv file2
cvs_file_path = 'https://raw.githubusercontent.com/openai/openai-cookbook/main/examples/data/AG_news_samples.csv'3
file_path = 'AG_news_samples.csv'4
5
if not os.path.exists(file_path):6
wget.download(cvs_file_path, file_path)7
print('File downloaded successfully.')8
else:9
print('File already exists in the local file system.')
In [5]:
1
df = pd.read_csv('AG_news_samples.csv')2
df
In [6]:
1
data = df.to_dict(orient='records')2
data[0]
Action Required
If you have a Free Starter Workspace deployed already, select the database from drop-down menu at the top of this notebook. It updates the connection_url to connect to that database.
Set up the database
Set up the SingleStoreDB database which will hold your data.
In [7]:
1
shared_tier_check = %sql show variables like 'is_shared_tier'2
if not shared_tier_check or shared_tier_check[0][1] == 'OFF':3
%sql DROP DATABASE IF EXISTS news;4
%sql CREATE DATABASE news;
Action Required
Make sure to select a database from the drop-down menu at the top of this notebook. It updates the connection_url to connect to that database.
In [8]:
1
%%sql2
DROP TABLE IF EXISTS news_articles;3
CREATE TABLE IF NOT EXISTS news_articles /* Creating table for sample data. */(4
title TEXT,5
description TEXT,6
genre TEXT,7
embedding BLOB,8
FULLTEXT (title, description)9
);
Get embeddings for every row based on the description column
In [9]:
1
# Will take around 3.5 minutes to get embeddings for all 2000 rows2
3
descriptions = [row['description'] for row in data]4
all_embeddings = model.encode(descriptions)5
all_embeddings.shape
Merge embedding values into data
rows.
In [10]:
1
for row, embedding in zip(data, all_embeddings):2
row['embedding'] = embedding
Here's an example of one row of the combined data.
In [11]:
1
data[0]
Populate the database
In [12]:
1
%sql TRUNCATE TABLE news_articles;2
3
import sqlalchemy as sa4
from singlestoredb import create_engine5
6
# Use create_table from singlestoredb since it uses the notebook connection URL7
conn = create_engine().connect()8
9
statement = sa.text('''10
INSERT INTO news.news_articles (11
title,12
description,13
genre,14
embedding15
)16
VALUES (17
:title,18
:description,19
:label,20
:embedding21
)22
''')23
24
conn.execute(statement, data)
Semantic search
Connect to OpenAI
In [13]:
1
import openai2
3
EMBEDDING_MODEL = 'text-embedding-ada-002'4
GPT_MODEL = 'gpt-3.5-turbo'
In [14]:
1
import getpass2
3
openai.api_key = getpass.getpass('OpenAI API Key: ')
Run semantic search and get scores
In [15]:
1
search_query = 'Articles about Aussie captures'2
search_embedding = model.encode(search_query)3
4
# Create the SQL statement.5
query_statement = sa.text('''6
SELECT7
title,8
description,9
genre,10
DOT_PRODUCT(embedding, :embedding) AS score11
FROM news.news_articles12
ORDER BY score DESC13
LIMIT 1014
''')15
16
# Execute the SQL statement.17
results = pd.DataFrame(conn.execute(query_statement, dict(embedding=search_embedding)))18
results
Hybrid search
This search finds the average of the score gotten from the semantic search and the score gotten from the key-word search and sorts the news articles by this combined score to perform an effective hybrid search.
In [16]:
1
hyb_query = 'Articles about Aussie captures'2
hyb_embedding = model.encode(hyb_query)3
4
# Create the SQL statement.5
hyb_statement = sa.text('''6
SELECT7
title,8
description,9
genre,10
DOT_PRODUCT(embedding, :embedding) AS semantic_score,11
MATCH(title, description) AGAINST (:query) AS keyword_score,12
(semantic_score + keyword_score) / 2 AS combined_score13
FROM news.news_articles14
ORDER BY combined_score DESC15
LIMIT 1016
''')17
18
# Execute the SQL statement.19
hyb_results = pd.DataFrame(conn.execute(hyb_statement, dict(embedding=hyb_embedding, query=hyb_query)))20
hyb_results
Clean up
Action Required
If you created a new database in your Standard or Premium Workspace, you can drop the database by running the cell below. Note: this will not drop your database for Free Starter Workspaces. To drop a Free Starter Workspace, terminate the Workspace using the UI.
In [17]:
1
shared_tier_check = %sql show variables like 'is_shared_tier'2
if not shared_tier_check or shared_tier_check[0][1] == 'OFF':3
%sql DROP DATABASE IF EXISTS news;
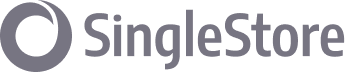
Details
About this Template
Hybrid search combines keyword search with semantic search, aiming to provide more accurate results.
This Notebook can be run in Shared Tier, Standard and Enterprise deployments.
Tags
License
This Notebook has been released under the Apache 2.0 open source license.
See Notebook in action
Launch this notebook in SingleStore and start executing queries instantly.