
IT Threat Detection, Part 3
Notebook

Note
This tutorial is meant for Standard & Premium Workspaces. You can't run this with a Free Starter Workspace due to restrictions on Storage. Create a Workspace using +group in the left nav & select Standard for this notebook. Gallery notebooks tagged with "Starter" are suitable to run on a Free Starter Workspace
Get pipeline data from Confluent (Kafka)
We recommend for that step to use a S1+ size workspace
Action Required
Make sure to select the siem_log_kafka_demo database from the drop-down menu at the top of this notebook. It updates the connection_url which is used by the %%sql magic command and SQLAlchemy to make connections to the selected database.
In [1]:
%%sqlDROP PIPELINE IF EXISTS `siem_log_real`;DROP TABLE IF EXISTS `siem_log_real`;
We start creating a simple table to load the logs into a JSON column
In [2]:
%%sqlCREATE TABLE IF NOT EXISTS `siem_log_real` (`logs` JSON COLLATE utf8_bin, SHARD KEY ()) AUTOSTATS_CARDINALITY_MODE=PERIODIC AUTOSTATS_HISTOGRAM_MODE=CREATE SQL_MODE='STRICT_ALL_TABLES';
We create a pipeline from the Confluent Cluster with an interval of 20ms
In [3]:
%%sqlCREATE PIPELINE `siem_log_real`AS LOAD DATA KAFKA 'pkc-p11xm.us-east-1.aws.confluent.cloud:9092/singlestore_topic'CONFIG '{\"sasl.username\": \"WTIVCYPLUAIMIAYQ\",\n \"sasl.mechanism\": \"PLAIN\",\n \"security.protocol\": \"SASL_SSL\",\n \"ssl.ca.location\": \"/etc/pki/ca-trust/extracted/pem/tls-ca-bundle.pem\"}'CREDENTIALS '{"sasl.password": "/qIOhlTFEK8RNNCc1qSOnpNj4mqhXfudBlQQFgRfc0qBEjfm99VcyvEuwPILBcnv"}'BATCH_INTERVAL 20DISABLE OFFSETS METADATA GCINTO TABLE `siem_log_real`FIELDS TERMINATED BY '\t' ENCLOSED BY '' ESCAPED BY '\\'LINES TERMINATED BY '\n' STARTING BY '';
Let's start the pipeline
In [4]:
%%sqlSTART PIPELINE siem_log_real;
We extract a few elements from the JSON column such as timestamp, Log_ID, and the vector to be stored in a blob format. Data is extracted as soon as an update is made to the table
In [5]:
%%sqlALTER TABLE siem_log_realADD COLUMN Timestamp as JSON_EXTRACT_STRING(`logs`,'Timestamp') PERSISTED datetime,ADD COLUMN model_res_blob AS JSON_ARRAY_PACK_F32(JSON_EXTRACT_STRING(`logs`, 'model_res')) PERSISTED BLOB,ADD COLUMN Log_ID AS JSON_EXTRACT_BIGINT(`logs`, 'Log_ID') PERSISTED bigint;
Install libraries for real-time dashboarding with Perspective
In [6]:
%pip install perspective-python --quiet
In [7]:
import perspectiveimport threadingimport randomimport timefrom datetime import datetime, datefrom perspective import Table, PerspectiveWidgetimport warningswarnings.filterwarnings('ignore')
We will set dashboard with a refresh rate of 500ms. We use two modes: stop and run to stop a dashboard retrieving results from the database.
In [8]:
def loop():while mode != 'stop':while mode == 'run':table.update(data_source())time.sleep(0.5)
Track Real-Time Connections
In [9]:
def data_source():result = %sql select Timestamp, count(*) as count_connections from siem_log_real group by Timestamp order by Timestamp desc limit 100result2 = list(result.dicts())return result2SCHEMA = {"Timestamp": datetime,"count_connections": int}
In [10]:
mode = 'run'table = perspective.Table(SCHEMA, limit=100)threading.Thread(target=loop).start()
In [11]:
perspective.PerspectiveWidget(table,title = "Track Real-Time Connections", group_by=["Timestamp"],plugin="Y Line",columns=["count_connections"])
In [12]:
mode = 'stop'
Monitor and Infer IT Threats using Semantic Search over Real-Time Data
In [13]:
def data_source():result = %sql WITH test_sql AS (SELECT Log_ID, TIMESTAMP, id, EUCLIDEAN_DISTANCE(model_res_blob, Model_Results) AS score,ROW_NUMBER() OVER(PARTITION BY Log_ID ORDER BY EUCLIDEAN_DISTANCE(model_res_blob, Model_Results) ASC) AS rn FROM (SELECT TIMESTAMP, Log_ID, model_res_blob FROM siem_log_real ORDER BY TIMESTAMP DESC LIMIT 20) CROSS JOIN model_results where score IS NOT NULL),label_table AS (SELECT Log_ID, TIMESTAMP,MAX(CASE WHEN id LIKE 'Bru%' OR id LIKE 'SQL%' THEN 'Malicious' ELSE 'Benign' END) as log_status FROM test_sql WHERE rn <= 100 GROUP BY Log_ID,TIMESTAMP order by TIMESTAMP DESC) SELECT log_status, count(Log_ID) as count_connections FROM label_table group by log_status;result2 = list(result.dicts())return result2SCHEMA = {"log_status": str,"count_connections": int}
In [14]:
mode = 'run'table = perspective.Table(SCHEMA, limit=100)threading.Thread(target=loop).start()
In [15]:
perspective.PerspectiveWidget(table,title = "Monitor Threat Inference", split_by=["log_status"],plugin="Y Line",columns=["count_connections"])
In [16]:
mode = 'stop'
Track latest connections with Inferences Threat Inference by Log IDs
In [17]:
def data_source():result = %sql WITH test_sql AS (SELECT Log_ID, TIMESTAMP, id, EUCLIDEAN_DISTANCE(model_res_blob, Model_Results) AS score,ROW_NUMBER() OVER(PARTITION BY Log_ID ORDER BY EUCLIDEAN_DISTANCE(model_res_blob, Model_Results) ASC) AS rn FROM (SELECT TIMESTAMP, Log_ID, model_res_blob FROM siem_log_real ORDER BY TIMESTAMP DESC LIMIT 20) CROSS JOIN model_results where score IS NOT NULL),label_table AS (SELECT Log_ID, TIMESTAMP,MAX(CASE WHEN id LIKE 'Bru%' OR id LIKE 'SQL%' THEN 'Malicious' ELSE 'Benign' END) as log_status FROM test_sql WHERE rn <= 100 GROUP BY Log_ID,TIMESTAMP order by TIMESTAMP DESC) SELECT * FROM label_table;result2 = list(result.dicts())return result2SCHEMA = {"Log_ID": str,"TIMESTAMP": datetime,"log_status": str}
In [18]:
mode = 'run'table = perspective.Table(SCHEMA, limit=20)threading.Thread(target=loop).start()
In [19]:
perspective.PerspectiveWidget(table,title = "Latest Connections", group_by=["TIMESTAMP"],plugin="Datagrid",columns=["count_attack"])
In [20]:
mode = 'stop'
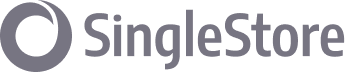
Details
Tags
License
This Notebook has been released under the Apache 2.0 open source license.