Rick Negrin
VP, Product Management
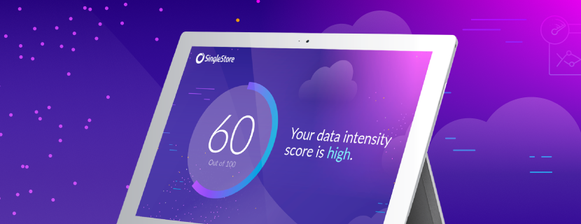
Data Intensity
How to Evaluate Your Application's Data Intensity with SingleStore's Data Intensity Assessment Calculator
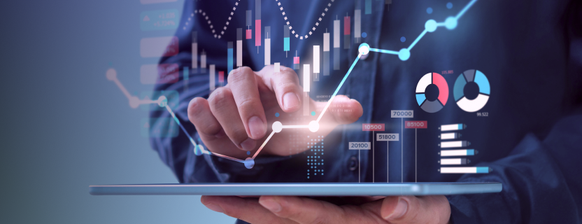
Product
Data Is Your Most Important Asset
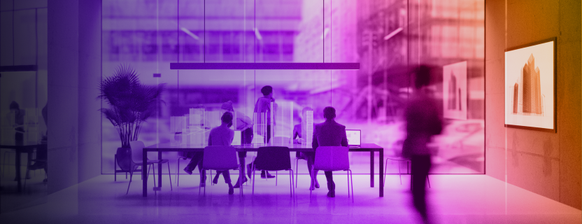
Product
Eliminating Database Sprawl, Part 2: How Three Companies Beat the Odds
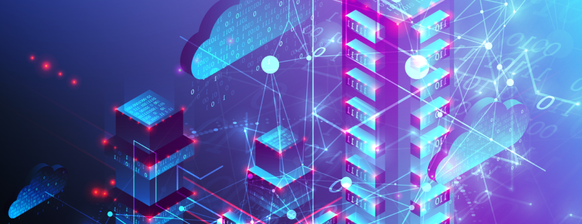
Product
Eliminating Database Sprawl, Part 1: How to Escape a Slow-moving Car Crash
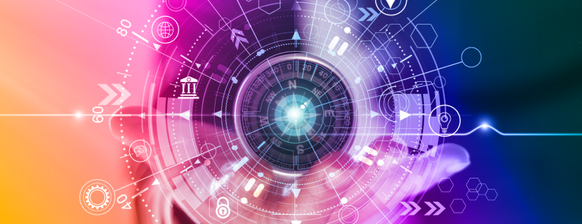
Product
Limitless Point-in-Time Recovery: What's in It for DBAs, Execs and Developers?
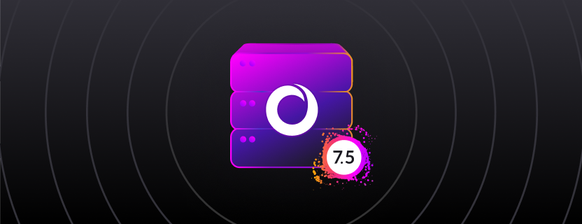
Product
SingleStoreDB Self-Managed 7.5 Now Available
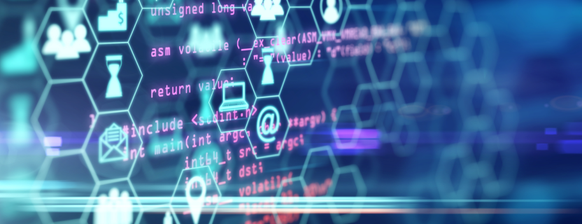
Data Intensity
Data-Intensive Applications Need A Modern Data Infrastructure
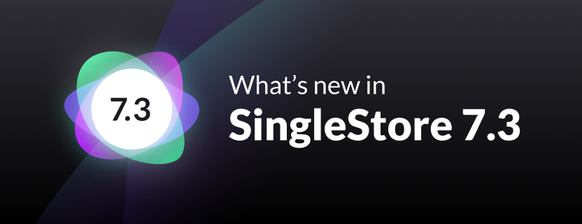
Product
SingleStoreDB Self-Managed 7.3 is Now Generally Available
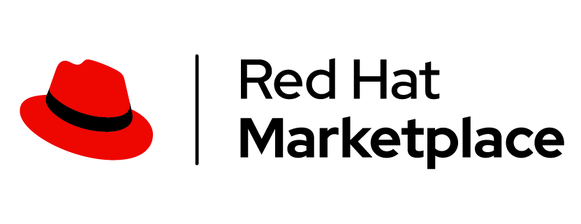
Product
SingleStore Now Available on Red Hat Marketplace
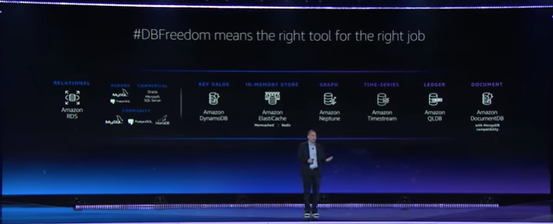
Data Intensity
Eliminate Data Infrastructure Sprawl, Stopping the Insanity
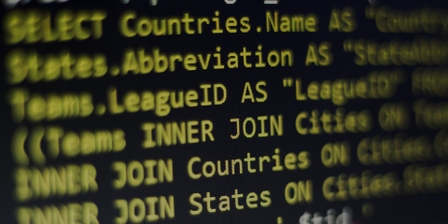
Data Intensity
NoSQL Databases: Why You Don’t Need Them
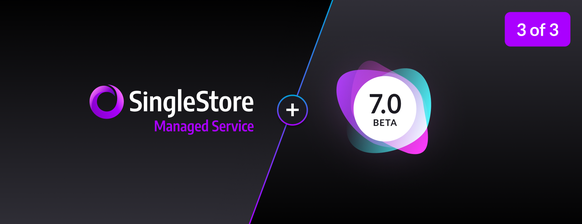
Product
Why SingleStore: SingleStoreDB Self-Managed 7.0 In Depth – Webinar Recap 3 of 3
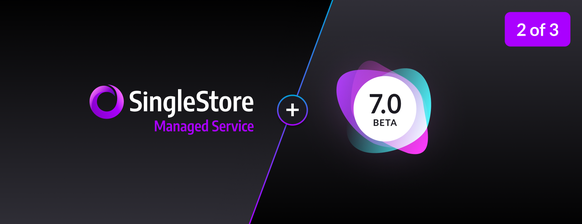
Product
Why SingleStore: SingleStore Helios In-Depth – Webinar Recap 2 of 3
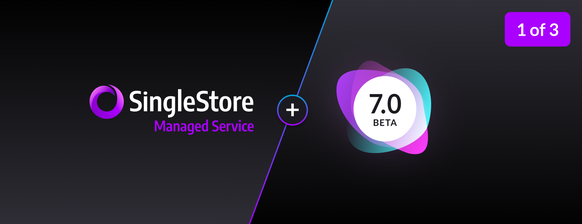
Product
Why SingleStore: SingleStore Database Overview – Webinar Recap 1 of 3
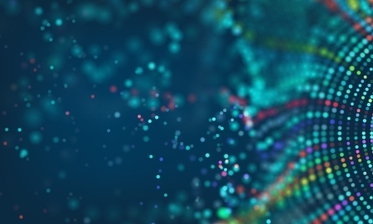
Data Intensity
The Need for Operational Analytics
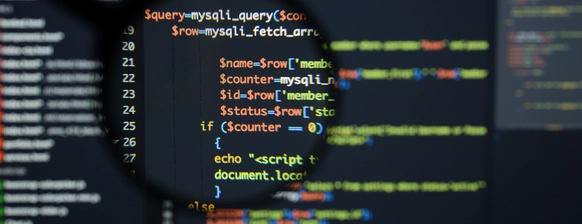
Data Intensity
Pre-Modern Databases: OLTP, OLAP, and NoSQL
Data Intensity
Selecting the Right Database for Your Scale Demands
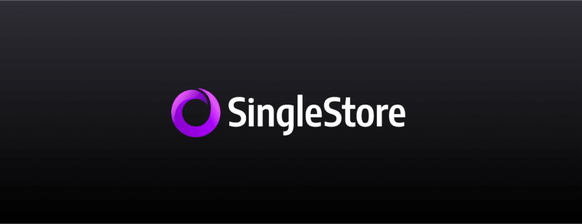
Product
Announcing SingleStoreDB Self-Managed 6.7: The No-Limits Database Gets Even Faster, Easier, and Now Free to Use
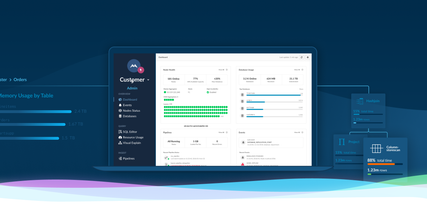
Product
A New Toolset for Managing and Monitoring SingleStore
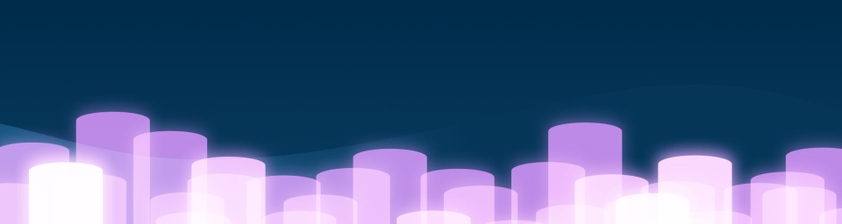
Product
Implementing a Scalable Multi-tenant Service
Showing 20 of 23 items