This post recaps a session at the SingleStore NOW 2024 Conference, held at the Chase Center on October 3, 2024. To view the entire session, check out the video at the bottom of the blog.
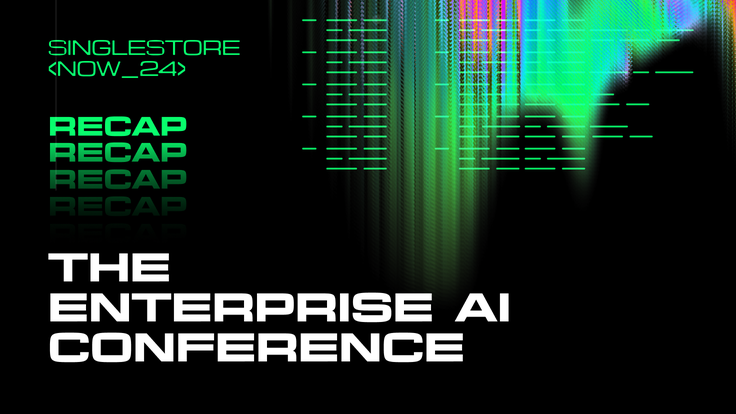
Overview
What does it take to build advanced knowledge assistants using Large Language Models (LLMs) and agentic reasoning? Jerry Liu of LlamaIndex shared his expertise on this subject — focusing on overcoming the limitations of traditional Retrieval Augmented Generation (RAG) setups, and how to build more sophisticated AI systems that can automate knowledge work and deliver high-value outputs.
About the speaker
As the Co-Founder and CEO of LlamaIndex, Jerry Liu leads a company focused on providing AI engineers with the tools and infrastructure needed to build generative AI applications. LlamaIndex is mainly known for its expertise in document parsing and building advanced knowledge assistants that integrate complex data types and agentic reasoning.
Key takeaways
Jerry's session outlined the core components needed to create a more powerful and production-ready knowledge assistant:
- Challenges with traditional RAG. Jerry highlighted the limitations of standard RAG architectures, where simple retrieval from vector databases combined with LLM responses often fails to handle complex queries. These systems can produce hallucinations and cannot reason through data, making them less effective for automating more advanced knowledge tasks.
- Building a high-quality data layer. A holistic data processing layer is the foundation of any effective knowledge assistant. Jerry emphasized the importance of accurate document parsing and indexing, especially when working with diverse and complex data types like PDFs, PowerPoint presentations and financial reports. LlamaIndex’s solution, LlamaParse, improves data extraction accuracy, reduces hallucinations and enables better responses from LLMs.
- Agentic reasoning for complex tasks. To move beyond simple question-and-answer setups, Jerry discussed the need for agentic reasoning, where LLMs can break down complex tasks into smaller components, use tools and iterate through a reasoning process. This approach enables the AI to tackle more intricate queries, like comparing documents or generating comprehensive reports — ultimately offering greater value to users.
- Advanced use cases and multimodal RAG. Jerry shared examples of how LlamaIndex enables users to build more complex applications, including multimodal RAG pipelines. These systems combine text and image inputs to deliver richer insights, making them particularly valuable for domains like financial analysis and internal reporting. He also noted use cases like customer support automation and financial report generation, where advanced RAG and agentic reasoning could significantly streamline workflows.
- Towards complete agentic systems. While the vision for fully autonomous AI agents is still emerging, Jerry emphasized the potential of these systems to automate higher-level decision-making processes. He discussed different architectures for integrating agents with RAG pipelines, from simple rule-based systems to more complex autonomous agents that can adjust their behavior based on the context and task at hand.
Take it to the next level
Ready to build a more advanced knowledge assistant for your enterprise? Access the full conference content to discover how tools like LlamaIndex and SingleStore can elevate your AI capabilities. Start your free SingleStore Helios® trial today to experience the power of real-time data processing and agentic AI applications.