Case Studies
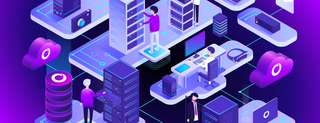
Case Studies
May 26, 2022Webinar Recap: The Future of Data Management: A Thought Leadership Chat
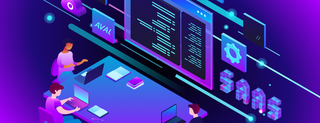
Case Studies
May 20, 2022How Three Developers Powered Their SaaS Apps with SingleStoreDB
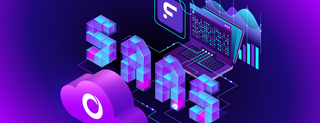
Case Studies
May 17, 2022Webinar Recap: Supercharging SaaS Applications With Foodics
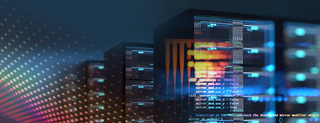
Case Studies
August 16, 2021How Three Companies Use SingleStore for Data Warehouse Augmentation
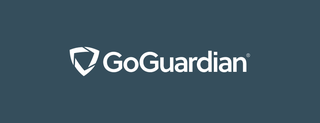
Case Studies
July 2, 2021GoGuardian’s Education Technology Platform, powered by SingleStoreDB Self-Managed, scales to serve over 18,000,000 students
Building a Gen AI App?
Build it on the database designed for AI applications.
Get started
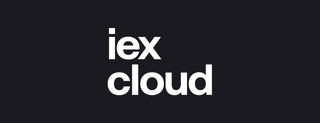
Case Studies
July 2, 2021IEX Cloud Processes Over 2.5B API Requests Daily with an 8ms Average Response Time Using SingleStore
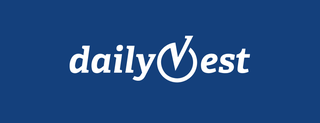
Case Studies
July 2, 2021How dailyVest Powers 401(k)s with SingleStore Helios and Improves Application Performance by 30%
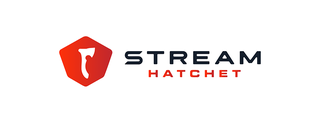
Case Studies
June 28, 2021How Stream Hatchet Powers a Real-Time Esports Analytics Platform with SingleStore Helios
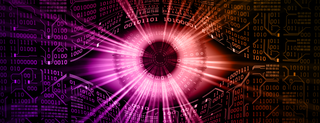
Case Studies
June 16, 2021Blink! It’s an Eternity for Data-Intensive Financial Services Applications
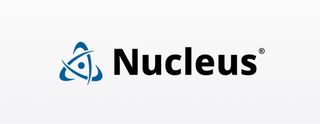
Case Studies
November 4, 2020Nucleus Security and SingleStore Partner to Manage Vulnerabilities at Scale
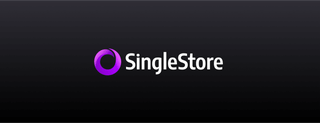
Case Studies
November 2, 2020SingleStore for Fastboards
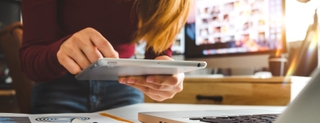
Case Studies
September 29, 2020Infosys and SingleStore: Working Together
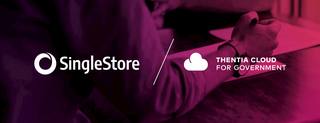
Case Studies
August 25, 2020SingleStore Powers the Rapid Rise of Thentia Regulatory Technology
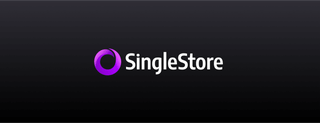
Case Studies
August 10, 2020How We Reverse-Engineered The Chicago Mercantile Exchange
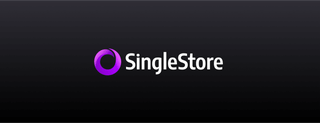
Case Studies
July 29, 2020Ventana Research Cites Data Infrastructure as Key to AI Success
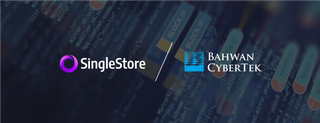
Case Studies
July 29, 2020Middle Eastern Technology Company Partners With BCT on Effort to Limit COVID-19 Spread using SingleStore
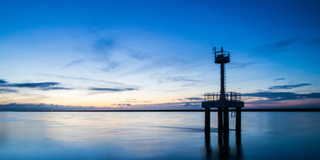
Case Studies
July 29, 2020SingleStore Ramps Up the Cadence of Financial Dashboards for an Industrial Machinery Leader
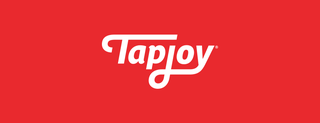
Case Studies
July 27, 2020Tapjoy Achieves 10X Performance Gains in Move to SingleStore
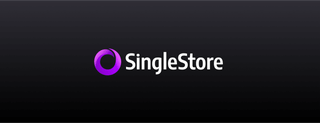
Case Studies
July 2, 2020Enabling Coronavirus Research with SingleStore and SafeGraph
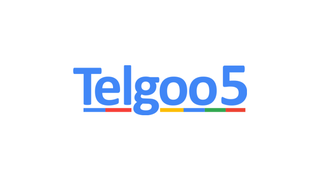
Case Studies
June 19, 2020Telgoo5 Uses SingleStore to Give MVNOs Ultra-Fast Connections to AT&T, T-Mobile, Verizon, and More
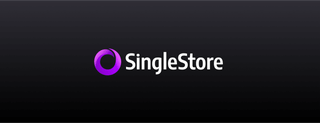
Case Studies
June 10, 2020SingleStore Powers True Digital Group’s Effort to Flatten the Curve of COVID-19
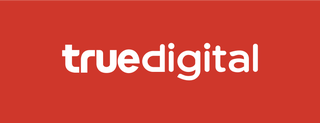
Case Studies
May 7, 2020Case Study: True Digital Group Helps to Flatten the Curve with SingleStore
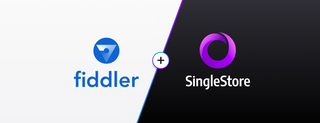
Case Studies
May 5, 2020Explainable Churn Analysis with SingleStore and Fiddler
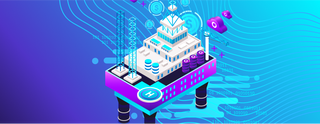
Case Studies
May 2, 2020SingleStore Improves Financial Operations for a Major Oil and Gas Company
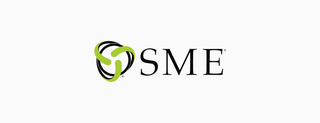
Case Studies
December 19, 2019Case Study: SingleStore Replaces Hadoop for Managing 2 Million New-Style Utility Meters
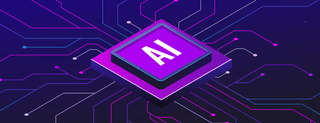
Case Studies
November 16, 2019Case Study: Moving to Kafka and AI at a Major Technology Services Company
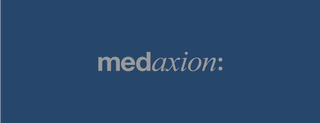
Case Studies
September 24, 2019Case Study: Medaxion Brings Real-Time Decision-Making to MedTech
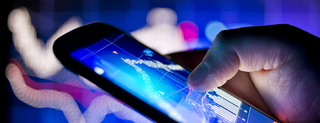
Case Studies
September 10, 2019Fiserv on Machine Learning & Real-Time Analytics at Financial Institutions
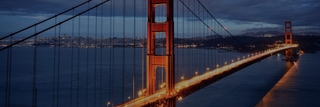
Case Studies
August 31, 2019Why Do Banks Need Real-Time Transaction Processing?
Showing 0 of 0 items