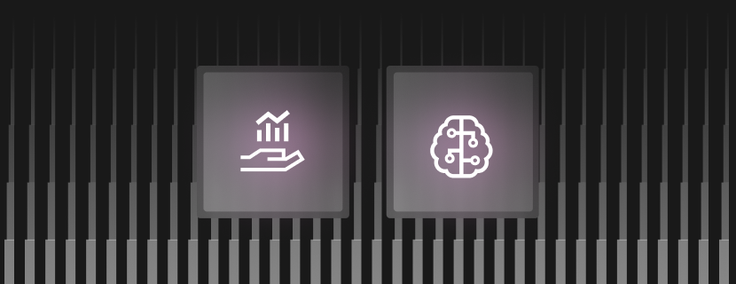
In this article, you'll learn more about the fundamentals of generative AI and how it can expand your customer analytics capabilities. When implemented, generative AI can lead to more accurate predictive models, targeted marketing campaigns and informed business strategies.
Amplifying Customer Analytics with Generative AI
To gain a competitive advantage in any market, understanding your customers' preferences, behaviors and needs is crucial.
Traditional customer analytic methods struggle to provide comprehensive insights because they can't capture complex patterns or generate realistic customer data. This can hinder accurate predictions, personalized marketing and decision making. However, generative artificial intelligence (AI), a cutting-edge branch of AI, provides a solution to these challenges.
Generative AI outperforms traditional data analytics by leveraging machine learning (ML) to generate synthetic customer data, expanding the data set for improved insights and personalized content. This ultimately boosts customer satisfaction, retention and revenue.
In this article, you'll learn more about the fundamentals of generative AI and how it can expand your customer analytics capabilities. When implemented, generative AI can lead to more accurate predictive models, targeted marketing campaigns and informed business strategies.
Why you need generative AI for customer analytics
Unlike traditional data analytics, which focuses on processing or analyzing existing information, generative AI utilizes the learning capabilities of neural networks. This ML algorithm identifies patterns in training data to make decisions on new data, simulating humanlike creative processes and generating new instances of data based on the probability distribution of the data.
The distinguishing feature of generative AI is its ability to produce synthetic content that is highly realistic and similar to what a human might create. Its ability to generate relevant data makes it a valuable tool for innovation, personalization and automation across fields such as creative arts, entertainment and healthcare.
In customer analytics, generative AI helps generate new content or insights that are related to real-world customer data, surpassing the limitations of historical information. It not only interprets existing data but actively contributes to data generation, enabling businesses to anticipate customer behaviors and needs more accurately.
For example, an eCommerce company that uses historical data to gain insights into customer behaviors would be unable to predict the impact of current events on future preferences. But with generative AI, the company can generate synthetic customer data that simulates current and future customer behavior based on existing data patterns.
This enables the discovery of new insights and trends that are more aligned with up-to-date customer behavior, helping the company adapt its marketing strategies, product recommendations and content creation to better meet the evolving customer needs and preferences.
Use cases for generative AI in customer analytics
When it comes to customer analytics, generative AI has diverse applications. Take a look at a few of them:
- Personalized customer experiences. Generative AI can create personalized customer experiences with tailored recommendations, content and interactions. It does this by analyzing individual preferences and behaviors as well as generating relevant and engaging content that resonates with individual customers.
- Customer segmentation and targeting. If you have limited data, generative AI can generate synthetic data points to augment your data set. It can create new but statistically credible customer information, helping to expand your potential customer segments (like data augmentation). This augmented data set can be used to perform a more robust analysis, personalized marketing emails, product descriptions and social media content that aligns with the interests and needs of each customer segment.
- Predictive analytics. Generative AI leverages pre-trained models to identify valuable patterns within limited data sets, enabling predictions about customer behaviors, preferences and future actions.
Challenges of traditional customer analytics, and how generative AI can help
To fully understand the impact of generative AI on customer analytics, take a look at some of the challenges you'll face with traditional analytics and how generative AI can help.
Data quality and quantity
With traditional analytics, the available customer data may be incomplete, outdated or inaccurate due to data decay, errors in data entry or lack of real-time updates. This can lead to inaccurate insights and bad decisions.
Additionally, privacy regulations can limit the collection of customer data, making it hard to adapt to changing customer behavior, especially when the data is processed in batches.
Generative AI utilizes data synthesis to expand the quality and quantity of data sets, improving the quality of analysis. These synthetic data points are credible simulations of real-world information, statistically matching expected characteristics and blending them with the existing data set to provide more information for analysis.
For instance, if a business has limited customer data for a new product launch, generative AI can generate synthetic profiles of potential customers, creating a more comprehensive view for market analysis and personalized targeting.
Biased data and models
If historical data used for analysis reflects existing biases in the real world, data bias can occur. For instance, a customer analytics model might conclude that women are more likely to engage with and convert through advertisements if the ads predominantly show beauty products. If the models are built on this data, they'll be biased. This can result in unfair or inaccurate predictions, impacting customer segmentation and recommendations.
Generative AI addresses bias in data and models by ensuring that the training data is diverse and representative of the population you're analyzing through data synthesis. It also implements bias mitigation techniques, such as resampling of underrepresented groups and adversarial training to reduce bias.
Interpretability and explainability
Sometimes, traditional models can provide results that are unexpected and difficult to explain or interpret. This is because its algorithm may be too simplistic to accurately model the data or capture irrelevant patterns (like underfitting or overfitting).
For instance, a recommendation system may suggest content to a user that is seemingly unrelated to their preferences without any logical understanding of why such a suggestion was made. This limitation can lead to difficulties in addressing bias and making informed decisions.
The diversity within generative AI data sets, along with the application of data augmentation and adversarial training techniques by generative AI models, generates outputs that are intuitive and logical.
Limited personalization
Limited personalization in customer analytics is usually a result of data limitations, lack of real-time insights and privacy regulations. Without the availability of sufficient and accurate data, achieving meaningful personalization with traditional customer analytics is difficult.
Competition and changing markets
In highly competitive markets, customer analytics must contend with saturation, where many businesses are vying for the same customer base. Traditional analytics may struggle to provide a competitive edge in such crowded spaces. Additionally, as markets evolve due to changing consumer trends and global events, traditional analytics models may not be able to adapt fast enough to provide timely insights and recommendations because of the fixed assumptions on which the algorithm was built.
Generative AI models provide up-to-date insights into changing markets by continuously adapting the model. Generative AI utilizes adversarial training to provide current customer preferences as new data becomes available. As the model adapts to changes in customer preferences and behaviors, it gives you more accurate predictions and personalized recommendations. However, this requires integration with data infrastructures, like SingleStoreDB, that offer the speed, scalability and flexibility you need to serve data in real time. This dynamic approach ensures that businesses stay tuned to customer needs, even as they evolve over time.
Customer expectations
Evolving customer expectations demand more personalized and relevant experiences. While traditional analytics may fall short in meeting these expectations due to its fixed model framework, generative AI utilizes adaptive learning to quickly capture evolving preferences and generate personalized content in real time, making it possible to respond to customer interactions and behavior as they happen.
Data from multiple sources
As markets diversify, data from various sources (like social media and IoT devices) becomes crucial. Traditional analytics have trouble integrating and analyzing data from diverse channels and are unable to provide robust insights due to the complexities and dimensionality of unstructured data. Generative AI incorporates data from heterogeneous data sources and handles diverse data complexities and dimensions for accurate predictive analytics.
Conclusion
Generative AI improves customer analytics by creating personalized customer experiences through data synthesis. It addresses challenges in traditional analytics by offering synthetic profiles and microsegmentation, and it enables improved decision making. Additionally, its anomaly detection goes beyond traditional methods, using contextual awareness to reduce false positives.
Utilizing generative AI for your customer analytics applications requires a data infrastructure that is robust, scalable and capable of handling both structured and unstructured data efficiently.
That's where SingleStoreDB can help. It provides a distributed architecture that helps users transact, analyze and contextualize data in real time. It also incorporates cutting-edge generative AI technology and optimized data architecture to deliver seamless and highly efficient data management that can readily integrate with generative AI tools like OpenAI, LangChain and LlamaIndex.
Sign up for a free cloud trial today to unlock the power of generative AI for your applications.
As customer expectations continue to change and market situations evolve, one thing is certain: the future of customer analytics is generative, and the possibilities are boundless.