All posts
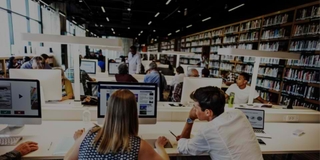
Case Studies
December 6, 2017Video: Delivering Real-Time Feedback Loops for Education
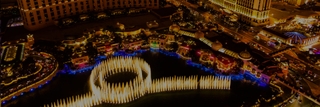
Trending
November 27, 2017Five Sessions to Attend at AWS re:Invent 2017
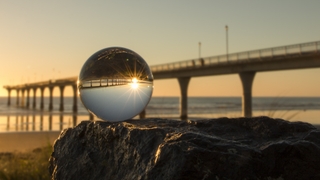
Trending
November 17, 2017Data Warehouse in the Age of AI Maturity
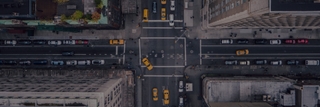
Data Intensity
November 8, 2017How Database Convergence Impacts the Coming Decades of Data Management
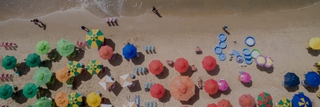
Engineering
October 27, 2017JSON Streaming And The Future Of Data Ingest
Building a Gen AI App?
Build it on the database designed for AI applications.
Get started
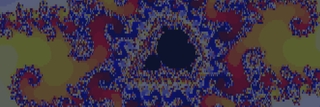
Engineering
October 19, 2017Running Stored Procedures on Distributed Systems with SingleStoreDB Self-Managed 6
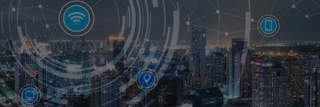
Data Intensity
October 13, 2017Why You Need a Real-Time Data Warehouse for IoT Applications
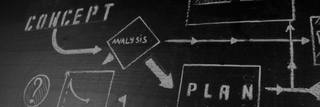
Data Intensity
October 6, 2017Designing for a Database: What’s Beyond the Query?
Product
September 26, 2017SingleStoreDB Self-Managed 6 Product Pillars and Machine Learning Approach
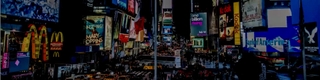
Trending
September 21, 2017Five Sessions to Attend at Strata Data Conference New York
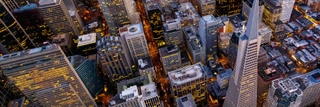
Trending
September 12, 2017Five Sessions to Attend at Join Conference in San Francisco
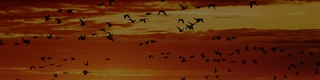
Trending
September 8, 2017Migrating from Traditional Databases to the Cloud
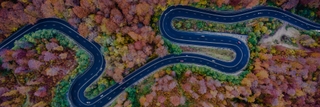
Trending
August 31, 2017Modern Data Warehousing, Meet AI
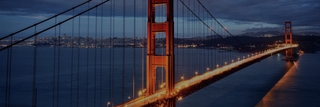
Trending
August 25, 2017Five Sessions to Attend at Kafka Summit San Francisco
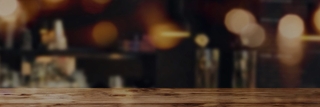
Data Intensity
August 18, 2017Real-Time Streaming, Analytics, and Visualization with SingleStore and Zoomdata
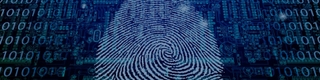
Trending
August 9, 2017Secure Real-Time Mission-Critical Analytics
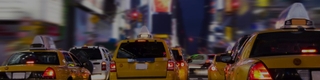
Case Studies
August 3, 20171.3 Billion NYC Taxi Rows into SingleStore
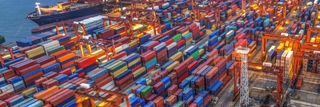
Engineering
July 26, 2017Psyduck: The SingleStore Journey to Containers
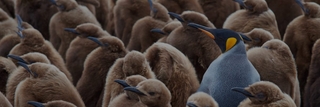
Engineering
July 19, 2017The Curious Case of Thread Group Identifiers
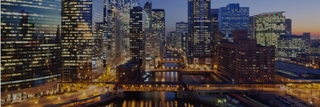
Trending
July 18, 20175 Sessions to See at AWS Summit Chicago
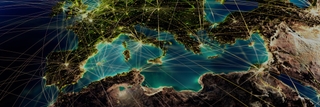
Data Intensity
July 14, 2017Delivering Scalable Self-Service Analytics
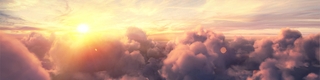
Data Intensity
July 10, 2017Key Considerations for a Cloud Data Warehouse
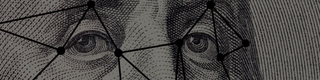
Engineering
June 29, 2017Image Recognition at the Speed of Memory Bandwidth
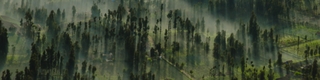
Product
June 16, 2017The Real-Time Data Warehouse for Hybrid Cloud
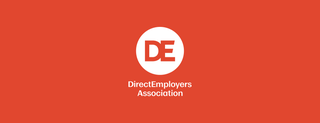
Case Studies
June 14, 2017Improving Recruiting Performance with Cloud-Based Real-Time Analytics
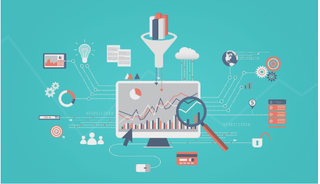
Data Intensity
June 6, 2017AI’s Secret Weapon: The Data Corpus
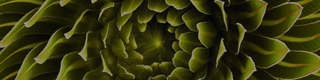
Company
June 2, 2017SingleStore Stands With Our Environment
Showing 0 of 0 items