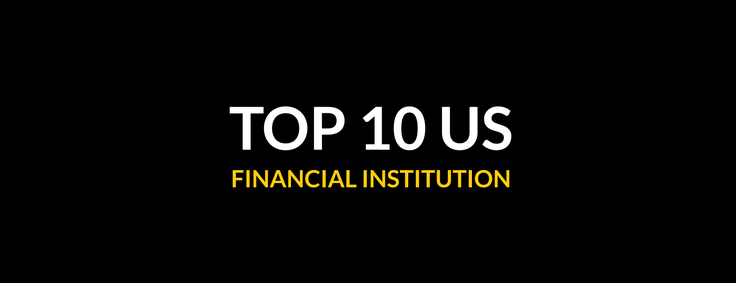
In this webinar Rick Negrin, Product Management VP at SingleStore, describes the importance of portfolio analytics, enhanced by machine learning models, to financial services institutions – helping them to meet customer needs and edge out competitors. He shows how SingleStore speeds up portfolio analytics at scale, with unmatched support for large numbers of simultaneous users – whether connecting via ad hoc SQL queries, business intelligence tools, apps, or machine learning models. You can view the recorded webinar and download the slides. He also describes how a major US financial services institutions implemented Kafka, Spark, and SingleStore, replacing Oracle and widespread use of cumbersome extract, transform, and load (ETL) routines, in this separate case study.
The business problem Rick discusses is the need to modernize portfolio analytics for reduced risk and better performance – both for the customer managing their portfolio, and for the institution offering portfolio management tools to customers. Institutional investors want smarter portfolio management services that deliver optimal returns while reducing their exposure to any one industry, currency, or other specific source of risk.
Portfolio managers want guided insights to help them avoid sudden or dramatic rebalancing of funds that can drive up costs and reduce confidence and customer loyalty. SingleStore powers a number of portfolio dashboards and what-if analysis, leveraging live market data for the most up-to-date view of the market. The separate case study shows how a major financial services company used SingleStore to solve these problems, supporting their leadership position in the market.
This webinar was originally presented as part of our webinar series, How Data Innovation is Transforming Banking (click the link to access the entire series of webinars and slides). This series includes several webinars, described in these three blog posts:
- Real-Time Fraud Detection for an Improved Customer Experience
- Providing Better Wealth Management with Real-Time Data
- Modernizing Portfolio Analytics for Reduced Risk and Better Performance (this webinar)
Also included are these two case studies:
- Replacing Exadata with SingleStore to Power Portfolio Analytics
- Machine Learning and Fraud Detection “On the Swipe” For Major US Bank
You can also read about SingleStore’s work in financial services – including use cases and reference architectures that are applicable across industries – in SingleStore’s Financial Services Solutions Guide. If you’d like to request a printed and bound copy, contact SingleStore.
The Role of the Database in Digital Transformation
Digital transformation remains the top priority by far for banks. This is confirmed by a Gartner study from 2019, but we at SingleStore hear it anecdotally in all the conversations that we have with financial institutions. This is because of the opportunity that digital transformation provides. When you to take advantage of new technologies, you can create new sources of revenue, and you can drive down your costs with new operating models, allowing you to deliver digital products and services that just weren’t possible before.
To make this happen, you need to have an architecture and operating platform that supports a new set of requirements. One need is to drive down latency: the time from when a new piece of information is born to the time you’re able to gain insight and take action on it. The effort is to get that as close to zero as possible.
When you do that, you can make faster data-driven actions in your business. So when something’s going on in the financial markets, the customer wants to understand it, to know what’s going on, as quickly as possible. And to be able to take action on it, in order to either reduce risk in a portfolio or perhaps take advantage of some new opportunity that’s come up.
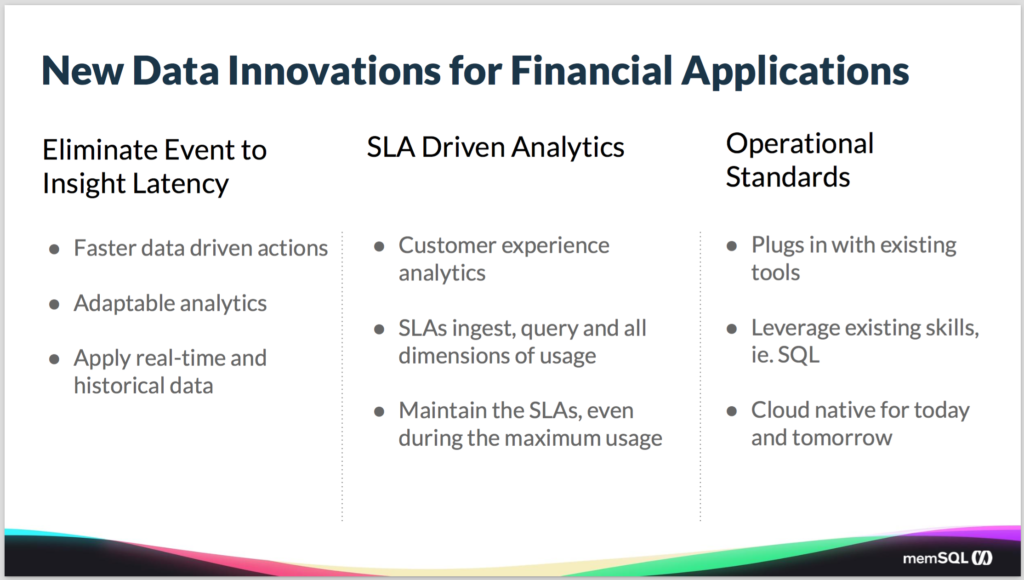
You also need adaptable analytics. The days of getting a static report once a week to your desk and then using that as information are far in the past. You need to be able to have an interactive experience with the data that’s flowing in. To be able to slice and dice it, looking at it across many different dimensions, to find the key insight that’s going to allow you to take advantage of what’s going on.
And you also want to be able to apply historical data in order to take the best advantage of what’s happening in real time in the market. This is especially important in the context of the machine learning algorithms that are being developed. Using the historical data to understand the patterns just so you can identify, given what you’re seeing in the market right now, what’s likely occurring, and how best to take advantage of it.
The second pillar is around service level agreements (SLAs), and particularly around analytics. So you are moving the systems from the back end, where you have maybe a couple of backend analysts who are working with the data, to the front end, where the people working with the data are the end users or the portfolio managers or even the end customers. The bar for the experience goes up dramatically. As does the need for concurrency – the need to support many simultaneous users, including those backend analysts but also BI tools and apps, at the same time.
You want interactive experiences that are snappy and responsive and allow you to get answers as quickly as possible. But to make that happen, you have to have SLAs on all the different dimensions of usage within the system, how fast the data is ingested into the system, how quickly you can query it out, how fast the storage is growing. You need SLAs across all those dimensions in order to guarantee a positive customer experience. And you need you to do that not just during the average load time, but you need to maintain those SLAs even at peak times.
Think of when some momentous event, or series of events, happens in the financial markets. You know, think 2008, or even 2000, and everybody’s coming in to use the system and you’ve got ten times more users concurrently trying to run their queries and trying to hit the storage system, the database system. You want to maintain those facilities even in the face of that – perhaps especially in the face of that. And then to do that, you need a system that can scale depending on the load.
And last, if you want a system that supports your operational standards, it should plug in with your existing tools so you can leverage all of the tool sets. This means robust support for ANSI SQL. And then of course, also the experience that your users have with those tools. So you don’t have to retrain all your users on how to operate and manage and optimize the system.
The more you can leverage the tools that you have, the easier it is to plug it into the overall ecosystem. And it’s got to be a system that’s not just built for today’s problems, but also for where everyone’s headed. And the place people are headed these days is all into cloud, into the public cloud systems. So it can’t be a legacy system, especially those that are tied to legacy hardware, because they won’t go where you’re headed. And you want something that is highly available and scalable and available to meet these requirements.
The Rise and Rise of Portfolio Analytics
Portfolio analytics is a huge market. It’s \$23 billion today, expected to grow to nearly double that in the next five years. What’s driving that is the combination of compliance, digitalization, and the drive to automate the backend processes. And as that happens it basically allows new opportunities in the market. It’s all coming from technological innovations that are happening in the fintech industry and providing a number of opportunities to go and take advantage of those technologies.
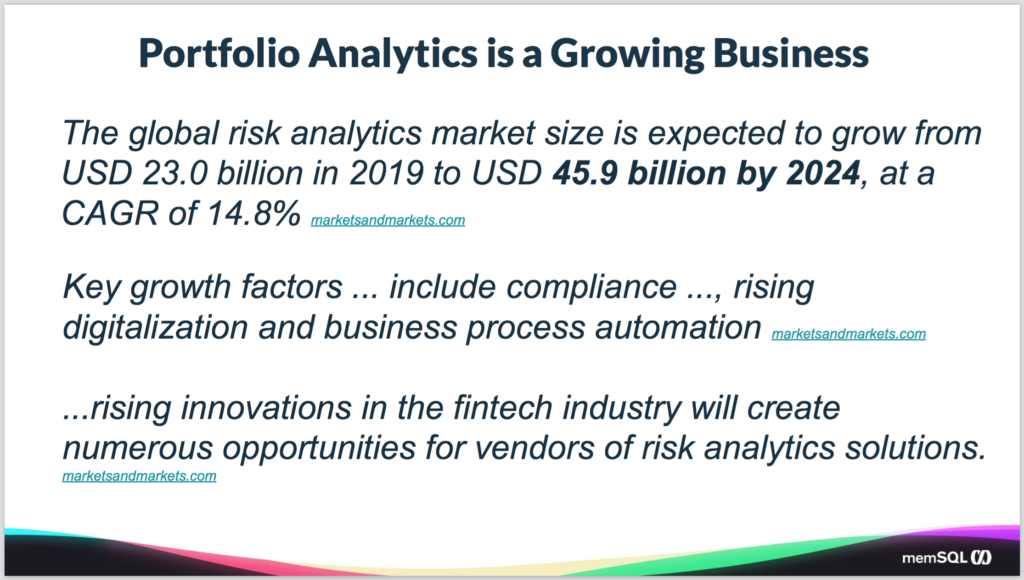
Now more concretely, what are the problems that financial services companies are facing? One is the need to combat passive investment vehicles. Those could become the default that people gravitate to because they’re easier and lower cost. You’re also seeing more competition among the large asset managers; it’s said that the number of asset managers has gone up by something like 10x over the last 20 years. There are more people trying to do asset management, and they are all using similar kinds of tools to do it.
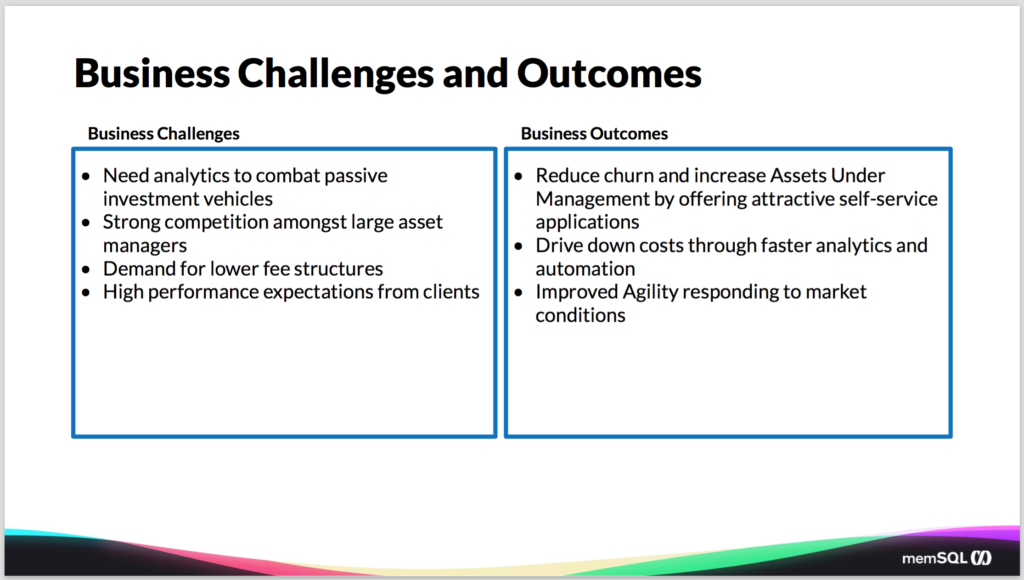
And then, because the passive investment vehicles have come to be so dominant, it’s driving down the fee structures, which means financial services companies need to be more cost effective and more cost efficient in how they operate. (For more on how one large financial services company met these requirements by moving from Oracle Exadata to SingleStore, see our case study – Ed.)
How SingleStore Powers Portfolio Analytics (and Digital Transformation Overall)
Now let’s go into why is SingleStore, why is it so good for portfolio analytics? Why did we build SingleStore, and how does it serve the market?
SingleStore is a cloud-native operational database built for speed and scale. But what we do is what we call operational analytics. So there are two common patterns in the database interchange. Two workloads that were the dominant patterns for a very long time. OLTP online transaction processing. Which is all about serving applications and being reliable and distributed and transactional and durable. And there’s OLAP – online analytical processing, also known as data warehousing. OLAP is all about serving analytical queries, complex joins, and aggregations. Separating the two is ETL – the extract, transform, and load process, to move data out of OLTP, remix it, and store it in OLAP. This means the OLAP world lived on stale data, with reporting and analytics mostly done, in an offline manner (despite the name), for people to make business decisions and for future plans.
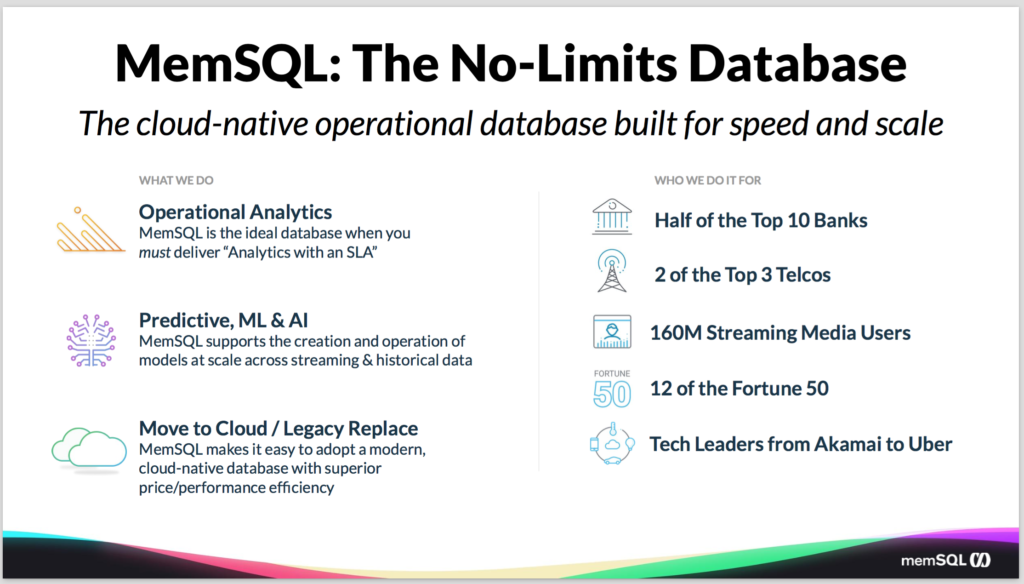
What operational analytics is, is it’s a third workflow that combines the requirements of the other two.
So it has all the analytical requirements in terms of the complexity of the queries, and the need for joins and aggregations, and time series and window functions and all the stuff you’d expect from a data technology. Combined with the requirements around maintaining an SLA, so it needs the reliability and availability and scalability of the operational systems. And when you have requirements that are part of both those things, SingleStore is the best database hands down.
So we boil this down into kind of a pithy statement of, when you need analytics with an SLA, SingleStore is the best fit. And this is the bread and butter of what we do with SingleStore and the kind of problems that we solve.
And we’re also seeing more and more people who are moving into doing more predictive ML and AI use cases. And it turns out what you need to solve those problems is a system that can operationalize your model at scale with a combination of historical and real time data. And it turns out SingleStore is a great fit for that. And so we see people doing more and more work in this space and it’s really just sort of the evolution of operational analytics.
A good example of this is we have a large bank in North America that’s built their credit card fraud system on top of SingleStore. And they chose it because the system they had before was too slow. And so they could catch the fraud, but only after it had happened – or even after it had happened several times. And then they would refund the money to the customer, but they would lose out.
What they wanted was a system that could identify and stop the fraud in its tracks before it happened. And to do that, you need to be able to load data continuously and in real time. Leverage historical data, for example, the user’s past purchasing history on the credit card. And then combine that with what happened with the credit card right now. And then make an instant decision around whether or not they’ll let the charge go through. And by leveraging SingleStore, they are able to do that. And using a custom model they built, they were able to implement that and achieve their objectives.
And the third pillar of what we do is around helping customers move from cloud and replace their legacy systems. Bust as customers, as everybody is moving to the public cloud, there’s a need to replace the legacy systems that won’t make it there. Either because they are using hardware that’s just not possible to take to the cloud. Or because they have legacy algorithms and technology and intellectual property that was built for slow-spinning disk 20 years ago and just are not applicable, or don’t make as much sense and don’t work as well in a cloud environment.
And SingleStore has the advantage of being a modern system built with modern data structures that works very well running in cloud environments. And giving you the availability and reliability and scalability of a cloud system. Combined with a front end that is familiar and easy to use, because it looks just like a regular relational database. So really giving you the best of both worlds, making it easy to move from legacy systems to something more modern that will run in the environment so you need to run. So that’s what we do.
And now who we do it for? As you may have surmised, finance is a top industry for us. Over half the top 10 banks in North America make use of SingleStore, and they do it not just for portfolio analytics, but for a number of other use cases like fraud that I mentioned, risk management and trade analytics and pretty much anything that fits that operational analytics workload. There are more and more use cases popping up all the time.
We have a number of SingleStore customers in the media space, Comcast and Pandora are just a couple of them. As well as in the telco space, like Verizon. Or people are doing things like tracking the quality of video or audio streaming. As well as doing user behavior analysis in order to implement things like personalization. As well as setting ad tracking and delivering analytics to partners like your advertisers, for how the ads are performing.
And the third key vertical for us is really in the high tech space. Everything from companies as big as Akamai and Uber making major investments to leverage SingleStore, to fast growing startups that need systems that can help maintain their growth as they’re building their customer-facing SaaS products.
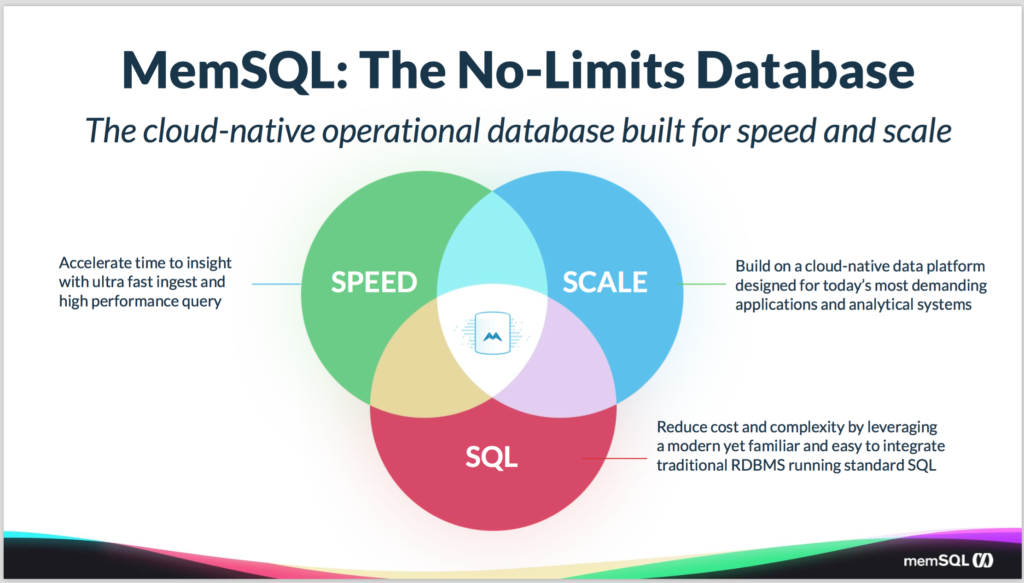
So the key problems that we solve are around speed and scale on SQL. So when you need speed, meaning bringing data in fast, pouring data out fast. Or performance matters, coupled with scale, or you need to be able to scale the system up as your usage and number of customers are growing. But you want to do it with a familiar interface that works with existing tools and technology and skillset of your people. When you have all three of those requirements, then SingleStore is the absolute best fit for you. Speed, scale, SQL, is how we describe our differentiation, the key pain points that we solve. And that’s what’s driving our business.
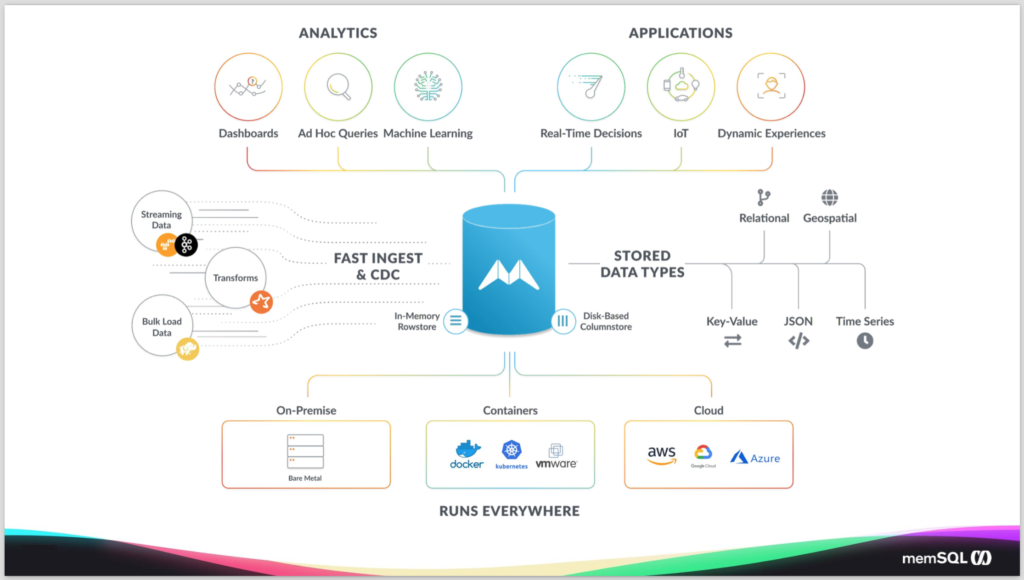
Now in terms of how we fit into the overall ecosystem, you can think of us like a regular relational database, and that data comes in from a data source. That data source can be standard operational legacy databases, could be streaming data sources like Spark and Kafka as I mentioned. Or bulk loading from big data systems.
And we have technologies that you bring data in from all those different sources regardless of the type or how you’re bringing it in. We have things like a CDC technology for bringing in data from operational systems. We have a feature called pipelines, so let’s you bring data in easily from Kafka and from data stores. And we have the ability to do transformations on the data so they can easily get it into the right shape before you put into the database. Now when you put it in the database, we have two different storage engines. We have in-memory rowstore and a disk-based columnstore.
We find customers tend to use a mix of the two. The rowstore tables are particularly good for hot data that needs to have a strict SLA, specifically when you do transactions or seeks. And the columnstore is much better for analytics and aggregation and the more analytical queries. And usually customers have the combination of the two on their system.
We support a number of different data types. So, whatever type of data you want to store. We can support it in SingleStore, obviously we handle the relational data, but we also have a native geospatial type. So you can easily index that and put it alongside of your relational data. We store a native JSON column type so you can store data in JSON or project out properties and easily index them and reference them within your SQL query.
We support time series and key value patterns as well. And then we also support a full text index, so you can pull text index elements of the database and reference that in your queries. And whether you’re using third-party analytical tools, like Tableau or Looker, Zoomdata or MicroStrategy, SingleStore supports all those. But many of our customers tend to use third party tools and many tend to do custom applications, or a mix, based on their needs and how they’re operating.
And of course in terms of how we run, you can run us on-premises, on bare metal. You can leverage VMs, or more recently, you can now leverage Kubernetes – we now have a Kubernetes operator. If you want to run us inside of a Kubernetes cluster on-prem or in the cloud, you can leverage our operator to easily deploy and manage SingleStore. And we run it across all the different cloud vendors – AWS, Google and Azure. We have customers who run us self-managed in all three. We also offer a managed service. If you don’t want to manage SingleStore yourself, we’ll do it for you.
How do we do all this? We do this through a set of features around having scalable SQL, so we have full ACID support. Meaning we support transactions and rollbacks combined with full ANSI SQL. So pretty much any SQL functions you would want supported in that SQL. And, as I mentioned, we have the full set of data types. Whether you’re storing documents or JSON, geospatial or full tech search, it’s all natively supported within the system.
We have fast ingest, and we have standard bulk load API, supporting the MySQL wire protocol. You can load data using files in CSV or other data types, other formats, bulk data is easily bulk loaded into the system. We have a native parallel stream ingest feature called Pipelines. It’s a first class object in the system. So you can easily load data in with the simple statement, CREATE PIPELINE. You point it at the Kafka queue or an S3 bucket or any of your favorite storage mechanisms and it immediately starts loading the data in a parallel. And we’re able to do this loading and queries simultaneously, because of the lock-free semantics within our system. Like I mentioned, we do use a different data structure under the covers that allows us to do this in a way that the legacy databases cannot.
Core Technologies that Power SingleStore Include MVCC, Skiplists
Pretty much all legacy databases were built on top of a technology called a b-tree. This was great in the days of slow spinning discs, when we needed to bring data back in chunks. But it came with certain locking semantics that made it very difficult to run a data query at the same time. It wasn’t a requirement in the original days or the early database.
SingleStore is built with a data structure called a skiplist. And with a skiplist, you can build them in a lock-free way. And that, combined with multi-version concurrency control (MVCC), our concurrency control system, allows us to be able to load data and to be able to query it simultaneously while it’s being loaded. This allows you to be streaming data in constantly, without blocking the system and preventing you from running queries. And this was one of the key underlying mechanisms that allows us to do what we do in a way that no one else can.
Under the covers, we’re a distributed, shared-nothing, massively parallel system. So when you put your data in the system it’s transparently charted across a number of modes. If you need more power, you can just add more nodes to the system. The system will automatically rebalanced the data, transparent to the developer or to the application. We do all this on commodity hardware. We don’t require any special hardware, you just need any machine that meets the minimum number of cores in memory and supports a modern version of Linux, you can be up and running with SingleStore.
We also provide high availability (HA), so it’s highly available, with transparent failover. So we’ll keep two copies of your data on two different machines at any given time. So if one of the machines dies, we transparently fail over and the application doesn’t even really notice. And we’re-cloud native. We sell software that you can deploy on-premises, and you can deploy us on any of the major cloud vendors. As I mentioned, we now have a Kubernetes operator, so that you can a run us in a Kubernetes cluster. So wherever you need to deploy we can make that work for you. And that’s it.
So at this point that’s the over view of SingleStore. Hope you learned something interesting. I’m happy to take questions.
Q&A
Q. What are some of the other use cases SingleStore is solving in financial services?
Great question. I think I may have touched on this earlier, but the portfolio analytics is definitely one of the primary ones. We also do things like trade analytics. So we have a large investment bank that wants to track each step in a trade process and identify any bottlenecks. So if there’s any bottlenecks they can immediately respond and go fix them, to make sure the trades are flowing as quickly as possible. So it’s something that they modeled within SingleStore, and they actually tried a number of different technologies. SingleStore is the only one that was able to meet their requirements for the number of updates per second they had to do. We’ve had a couple of other customers who’ve also implemented trade analytics that way.
Risk management is another use case where you want to keep track of how much risk you’ve taken on during the day. In the past, often larger, especially investment banks can only tell how much risk they had taken on by doing a report at the end of the day. They try to leave quite a bit of buffer in order to make sure they didn’t take on too much risk and then violate the FTC rules. And so by having that available to them in real time, they can be much more specific and precise about how much risk they’re taking on. And not have to leave any opportunities on the table because they’re in fear of possibly going over a line that they might not be actually going over.
And then one of the other use cases I mentioned was fraud. We actually have a fair amount of fraud use cases where people are tracking down either credit card fraud or other forms of fraud. But yeah, we have more use cases popping up all the time.
Q. Can we get some help if we want to do a proof of concept with SingleStore?
Absolutely. We have a number of highly trained sales engineers. We’ll be happy to help you get up and running and give you sort of the best practices. And help make sure that your system is optimally tuned to achieve the performance and scale you require.
Q. What’s coming in the future for SingleStore?
Great question. So we have a lot of good stuff, but there’s a lot more to go build. So we’re making additional investments and more on the transactional side. Particularly on recovery from disaster. Today we support backups and the backups are now online operations. So you can run that and it won’t block your system. There are full backups. So we’ve had a number of requests, like can we do incremental backups. Which allow you to run backup more often, reduce your RPO. So that’s a feature going to be coming soon.
As well as things like point in time restore. So you can restore back to a particular point in time, again to try to reduce that RPO to as little as possible.
We’re making efforts around simplifying the choices people have to make. So I mentioned we have a rowstore and a columnstore. And so having both of those in one system, with SQL queries able to span the two types of tables, is a huge innovation, and hugely valuable to customers. But it then presents a decision point when you’re designing your app around, “Hey, how do I decide whether I should put this data in rows or in columns? Make this table rowstore or columnstore?” And so we’re working on merging the rowstore and columnstore technologies together in something we call Universal Storage, to allow you to just have one table type, say CREATE TABLE, and you don’t have to think about how the data is stored underneath. SingleStore takes care of that and organizes it for you.
And then the third pillar of investment is around the managed service, which I mentioned is currently a private preview. But we’ll be taking that into public preview soon. And we’re making investments to automate the management to the system to make it easy for you to be able to just spin up a cluster and you use it in SingleStore. Without having to worry about the physical management and troubleshooting characteristic.
We invite you to learn more about SingleStore at www.singlestore.com, or give us a try for free it singlestore.com/cloud-trial/.