Posts by
Floyd Smith
Director, Content Marketing
FS
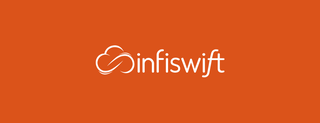
Data Intensity
August 19, 2020How Infiswift Supercharged Its Analytics for IoT & AI Applications
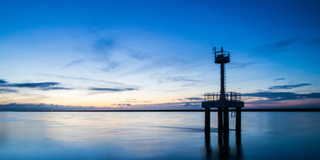
Case Studies
July 29, 2020SingleStore Ramps Up the Cadence of Financial Dashboards for an Industrial Machinery Leader
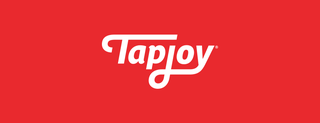
Case Studies
July 27, 2020Tapjoy Achieves 10X Performance Gains in Move to SingleStore
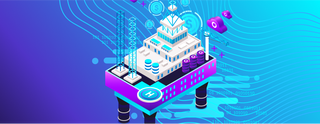
Case Studies
May 2, 2020SingleStore Improves Financial Operations for a Major Oil and Gas Company
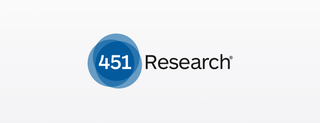
Trending
April 2, 2020A HOAP-ful New Perspective from 451 Research
Building a Gen AI App?
Build it on the database designed for AI applications.
Get started
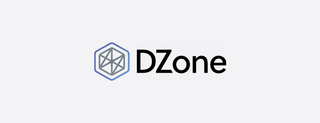
Trending
March 11, 2020Cloud Database Trend Report from DZone Features SingleStore
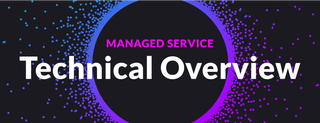
Product
March 5, 2020SingleStore Helios Technical Overview
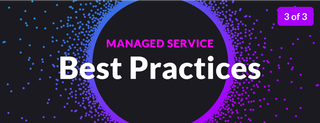
Product
March 1, 2020Best Practices for Migrating Your Database to the Cloud – Webinar Recap 3 of 3
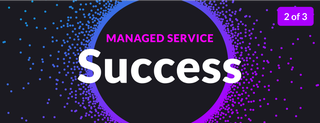
Product
February 28, 2020Ensuring a Successful Cloud Data Migration – Webinar Recap 2 of 3
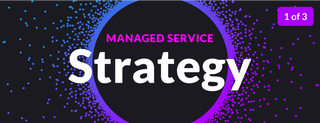
Product
February 27, 2020Migration Strategy for Moving Operational Databases to the Cloud – Webinar Recap 1 of 3
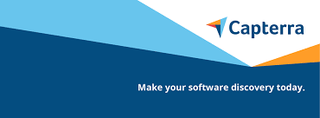
Data Intensity
February 12, 2020Capterra Captures Positive Reviews of SingleStore
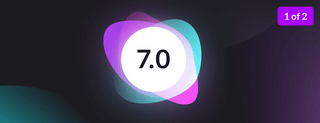
Engineering
February 8, 2020Building Fast Distributed Synchronous Replication at SingleStore – Webinar Recap 1 of 2
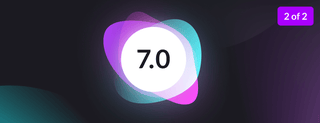
Engineering
February 8, 2020Building Fast Distributed Synchronous Replication at SingleStore – Webinar Recap 2 of 2
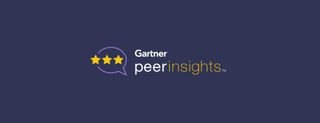
Data Intensity
January 27, 2020Gartner Peer Insights Applauds SingleStore
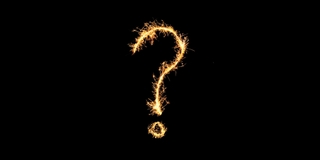
Data Intensity
January 10, 2020New Year, New FAQs
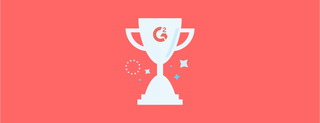
Data Intensity
January 9, 2020The G2 Crowd Has (Much) More to Say About SingleStore
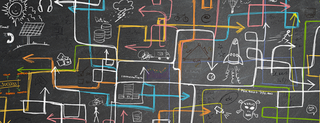
Engineering
January 2, 2020The Write Stuff
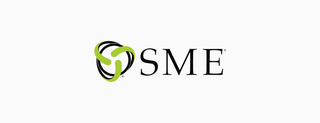
Case Studies
December 19, 2019Case Study: SingleStore Replaces Hadoop for Managing 2 Million New-Style Utility Meters
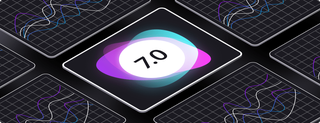
Product
December 18, 2019Webinar: Time Series Data Capture & Analysis in SingleStoreDB Self-Managed 7.0
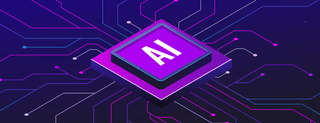
Case Studies
November 16, 2019Case Study: Moving to Kafka and AI at a Major Technology Services Company
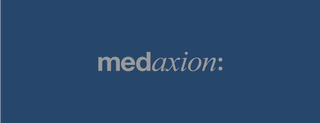
Case Studies
September 24, 2019Case Study: Medaxion Brings Real-Time Decision-Making to MedTech
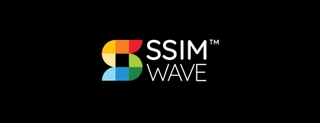
Data Intensity
September 24, 2019Case Study: Emmy-Winning SSIMWAVE Chooses SingleStore for Scalability, Performance, and More
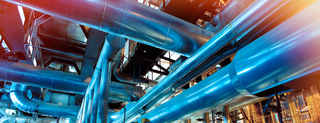
Product
September 1, 2019CREATE PIPELINE: Real-Time Streaming and Exactly-Once Semantics with Kafka
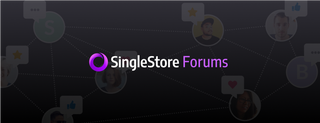
Company
September 1, 2019Community Stars Light Up SingleStore Forums
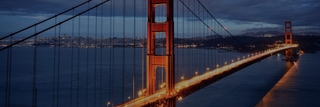
Case Studies
August 31, 2019Why Do Banks Need Real-Time Transaction Processing?
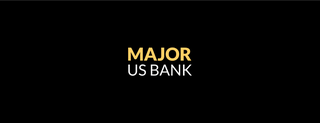
Case Studies
August 25, 2019Case Study: Fraud Detection “On the Swipe” For a Major US Bank
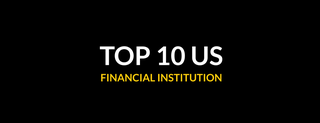
Data Intensity
August 24, 2019Webinar: Modernizing Portfolio Analytics for Reduced Risk and Better Performance
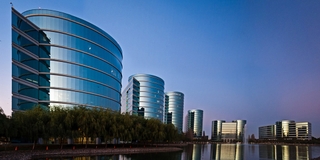
Data Intensity
August 24, 2019Case Study: Replacing Exadata with SingleStore to Power Portfolio Analytics and Machine Learning
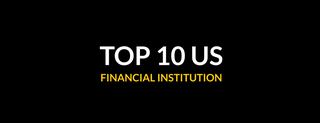
Data Intensity
August 22, 2019Webinar: Real-Time Fraud Detection for an Improved User Experience
Showing 0 of 0 items