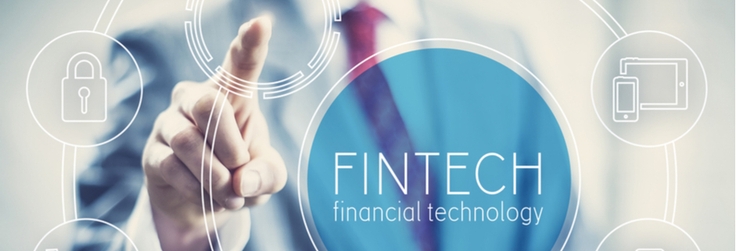
In this webinar, SingleStore Product Marketing Manager Mike Boyarski describes trends in data initiatives for banks and other financial services companies. Data is the lifeblood of modern financial services companies, and SingleStore, as the world’s fastest database, is rapidly growing its footprint in financial services.
Financial services companies are leaders in digital transformation initiatives, working diligently to wring business value from the latest initiatives. According to Gartner’s 2019 CIO Agenda survey, Digital transformation is the top priority for banks, followed by growth in revenue; operational excellence; customer experience; cost optimization/reduction; and data and analytics initiatives (which are also likely to show up in the “digital transformation” bucket). As Gartner puts it, “… the digital transformation of banks creates new sources of revenue, supports new enterprise operating models and delivers digital products and services.”
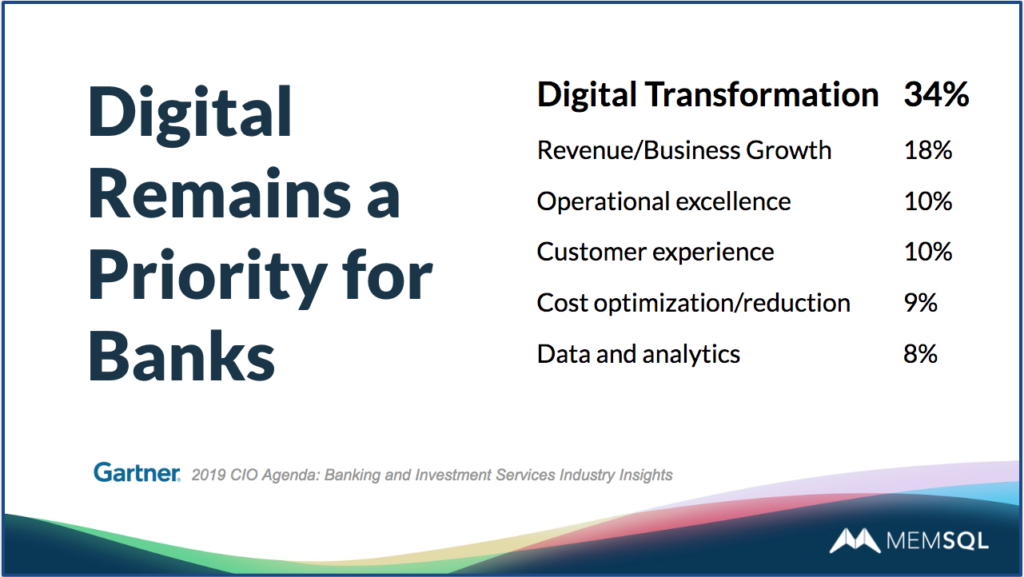
We encourage you to review these highlights, then view the webinar recording for Mike’s in-depth treatment of this important topic.
Banks Bring Digital Transformation to Life
The key data initiatives that banks are pursuing as part of digital transformation include:
- Premium customer experiences. Areas such as wealth management, always-on advisory services, and personalization are receiving focused attention to improving the customer experience.
- Smart risk management. Areas of interest include automating compliance reporting, risk management analysis carried out before a trade is made, and governance for big data, as well as greater responsiveness for risk management analysis.
- Cloud computing. Cloud initiatives include agile machine learning (ML) and AI services, elastic computing and storage, and hybrid architectures across cloud providers and on-premises data processing.
- AI/ML. Machine learning and AI are being used for fraud detection (often, in real time rather than in daily batch analysis), “robo advisors” that use computer-based intelligence to provide trading and investment advice, and portfolio analysis, including for risk management.
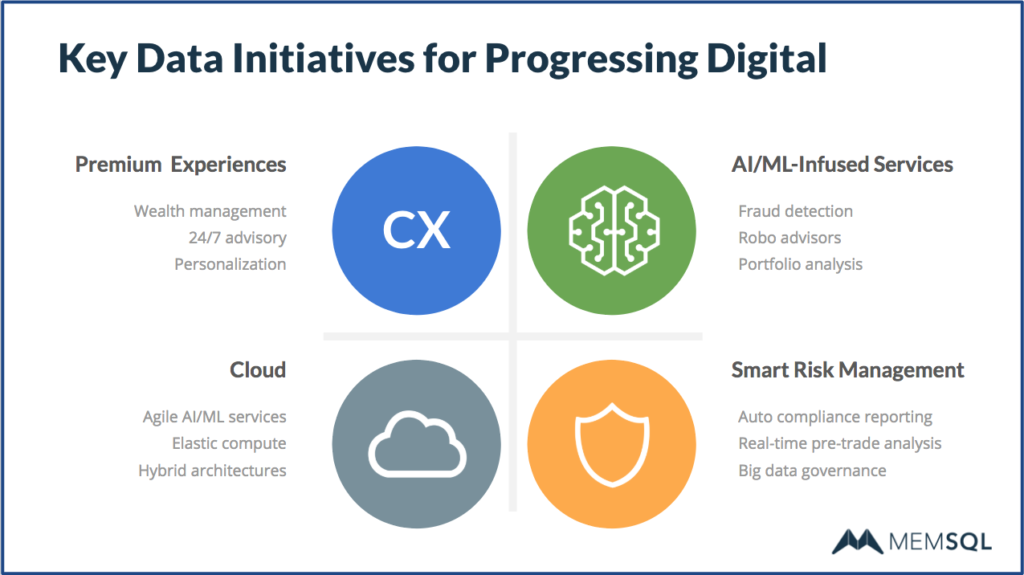
How do data innovations support improvements to banking applications? Mike cites the elimination of latency between real-world events, such as a stock trade, and actionable insights, such as offering relevant services; faster data architectures for greater agility; and reducing operational burdens, even while improving responsiveness and concurrency.
How Does SingleStore Help?
SingleStore helps to meet requirements that are commonly found in banking:
- Single-node database augmentation. Banks are often looking to improve performance for legacy, single-node relational databases such as Oracle, Sybase, and Netezza.
- Hadoop acceleration. Hadoop/HDFS implementations are often slow and hard to query, even when additional layers such as Hive and Spark are used to try to improve performance. SingleStore provides scalable SQL for performance and usability.
- Path to cloud. As a cloud-native database that also excels in on-premises deployments, SingleStore provides a path for banks to innovate while keeping the door open between cloud and on-premises use.
- Real-time AI/ML. As machine learning and AI move from research to deployment, scalable SingleStore has the capacity, concurrency support, and performance to deliver business value.
In particular, banks have strong needs in both of the data table types that mark different use cases in data management. SingleStore is unique in performing strongly on both while offering flexibility between the two:
- Row-oriented tables (often memory-based). Frequently updated data tables and transactions commonly run in row-oriented tables, as do data with critical timing requirements for accessibility. (Think device control, as in IoT.)
- Column-oriented tables (usually disk-based). Less frequently updated data tables, often featuring different data aggregations and sort orders for stellar query performance. These tables tend to have high concurrency requirements. (Think business intelligence, operational databases for app support, and operational AI/ML.)
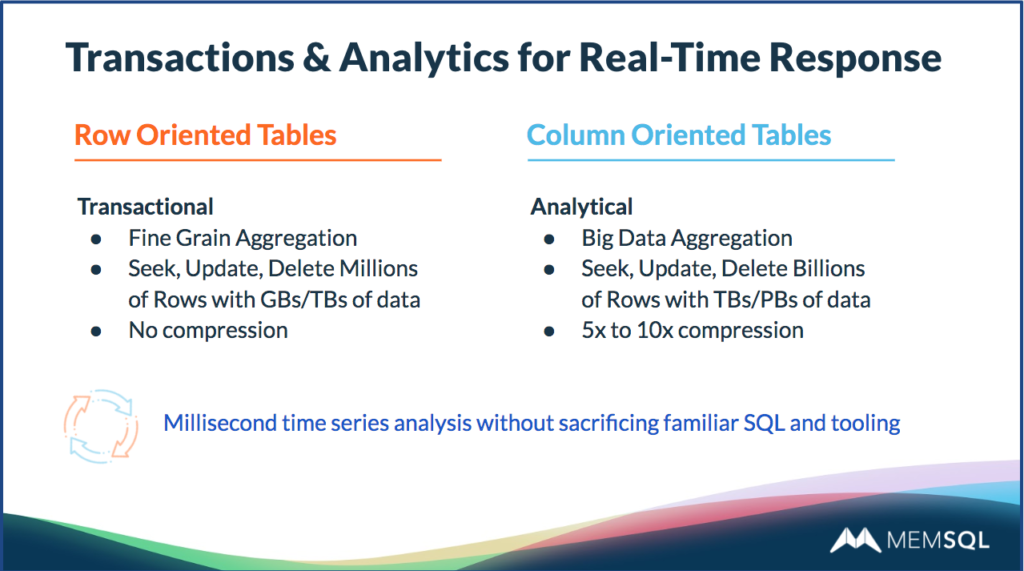
Q&A
Q. How does SingleStore work with Apache Spark and what use cases does it support?
A. Spark is great at doing acceleration for your Hadoop architecture, but in terms of how it works with SingleStore, we see folks using it in two ways: they use it for transforming data in the stream and for using the ML library for processing the data before it lands in SingleStore. We see Spark used more as a transformation layer, especially for the ML logic.
Q. How is SingleStore used for geospatial support?
A. This is crucial functionality for several users, and Uber has really led the way in taking advantage of our geospatial support (see the Uber engineering blog, view a live video presentation, and visit our customers page – Ed.). But for details, let me refer you to the SingleStore documentation.
Q. Can SingleStore work as a dedicated data warehouse, like Netezza?
A. SingleStore definitely can work that way. But SingleStore is a unique technology. We provide an ingest engine that’s largely rowstore based, and in memory based. That’s the capability that traditional data warehouses just don’t have. We absolutely can also function as a dedicated data warehouse, and we have customers using us for that. We look forward to publishing some benchmarks to show just how fast we are at these functions.
Following Up
Intrigued? It’s easy to learn more.
Read the case studies referred to in the webinar – wealth management dashboards and portfolio risk management.
View the webinar, including Q&A, and download the slides here.