All posts
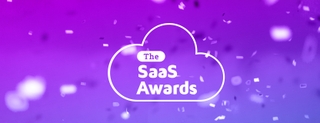
Company
August 25, 2020SingleStore Named 2020 SaaS Awards Winner
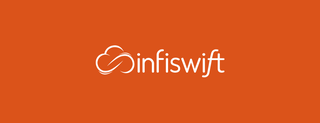
Data Intensity
August 19, 2020How Infiswift Supercharged Its Analytics for IoT & AI Applications
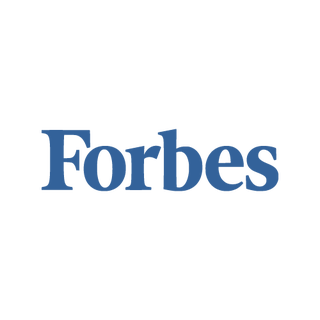
Trending
August 12, 2020Digital Twins: An Important Next Step In The Data Economy
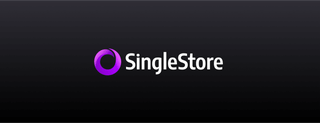
Case Studies
August 10, 2020How We Reverse-Engineered The Chicago Mercantile Exchange
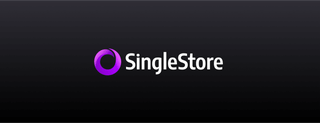
Company
August 10, 2020SingleStore Shortlisted as a Finalist in the 2020 SaaS Awards
Building a Gen AI App?
Build it on the database designed for AI applications.
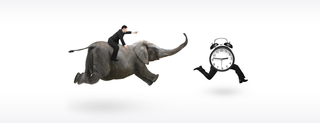
Data Intensity
August 6, 2020Hadoop: The Chronicle of an Expected Decline
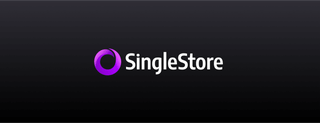
Case Studies
July 29, 2020Ventana Research Cites Data Infrastructure as Key to AI Success
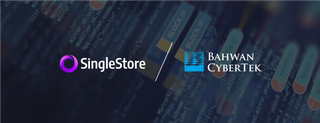
Case Studies
July 29, 2020Middle Eastern Technology Company Partners With BCT on Effort to Limit COVID-19 Spread using SingleStore
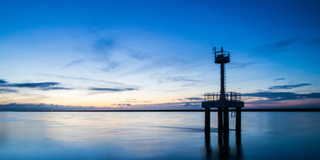
Case Studies
July 29, 2020SingleStore Ramps Up the Cadence of Financial Dashboards for an Industrial Machinery Leader
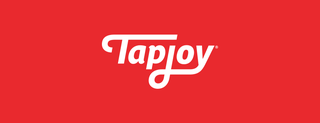
Case Studies
July 27, 2020Tapjoy Achieves 10X Performance Gains in Move to SingleStore
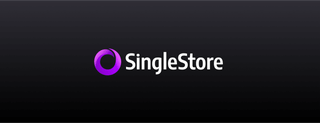
Trending
July 22, 2020SingleStore Establishes Commitment to Latin American Market with LATBC Strategic Partnership
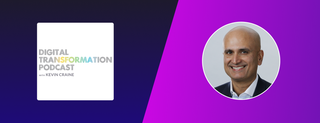
Trending
July 22, 2020Digital Transformation Podcast: 10 Key Insights into Business in the COVID-19 Era
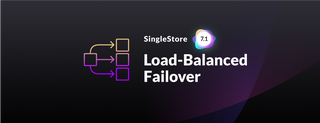
Product
July 16, 2020Load-Balanced Failover in SingleStoreDB Self-Managed 7.1
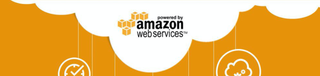
Trending
July 15, 2020SingleStore Expands Collaboration With Amazon Web Services, Joins ISV Workload Migration Program
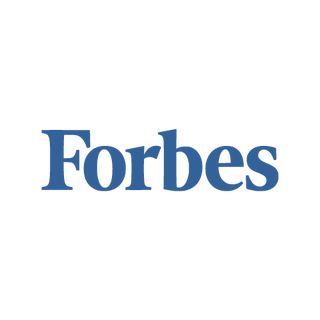
Data Intensity
July 13, 2020Why We Need Management And Scalability To Benefit From The Power Of Data
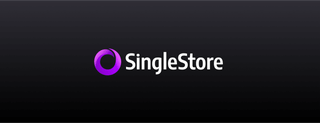
Case Studies
July 2, 2020Enabling Coronavirus Research with SingleStore and SafeGraph
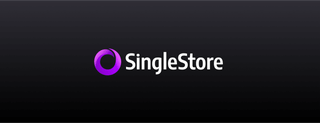
Product
July 1, 2020SingleStore Introduces a New UI for Installing SingleStore Clusters
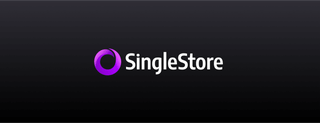
Company
June 30, 2020SingleStore Names Former Google BigQuery Leader Tigani as Chief Product Officer
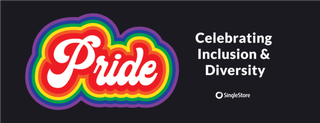
Company
June 30, 2020Celebrating Pride Month, a Great Innovator, and Understanding Where AI Stands Today
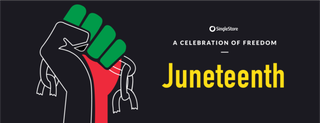
Company
June 26, 2020Keeping the Discussion and Movement Going: Diversity and Inclusion at Work
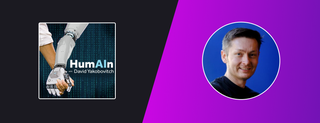
Data Intensity
June 24, 2020Podcast: The Importance of Data Management and AI During COVID-19 with Nikita Shamgunov
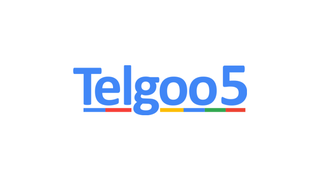
Case Studies
June 19, 2020Telgoo5 Uses SingleStore to Give MVNOs Ultra-Fast Connections to AT&T, T-Mobile, Verizon, and More
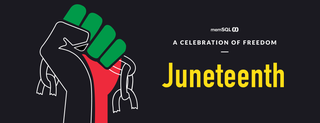
Company
June 17, 2020Now Is the Time for All of Us to Learn, Reflect, and Take Action
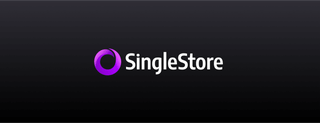
Case Studies
June 10, 2020SingleStore Powers True Digital Group’s Effort to Flatten the Curve of COVID-19
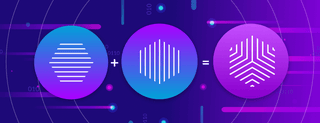
Data Intensity
June 10, 2020What is HTAP?
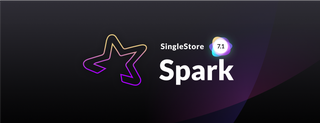
Product
June 10, 2020SingleStore Introduces New Spark Connector 3.0
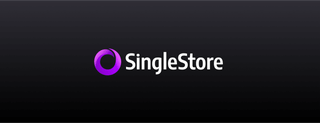
Data Intensity
June 8, 2020Why We Need Management and Scalability to Benefit from the Power of Data
Showing 0 of 0 items